Documents
Presentation Slides
Distributed Estimation of Latent Parameters in State Space Models Using Separable Likelihoods
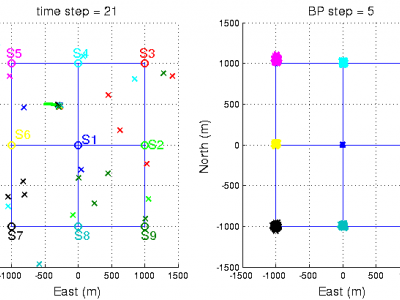
- Citation Author(s):
- Submitted by:
- Murat Uney
- Last updated:
- 22 March 2016 - 4:44pm
- Document Type:
- Presentation Slides
- Document Year:
- 2016
- Event:
- Presenters:
- Murat Uney
- Paper Code:
- 4171
- Categories:
- Keywords:
- Log in to post comments
This work is a part of our research on scalable and/or distributed fusion and sensor calibration. We address parameter estimation in multi-sensor state space models which underpins surveillance applications with sensor networks. The parameter likelihood of the problem involves centralised Bayesian filtering of multi-sensor data, which lacks scalability with the number of sensors and induces a large communication load. We propose separable likelihoods which approximate the centralised likelihood with single sensor filtering terms. We provide bounds on the approximation error and highlight that the quality of approximation is related to the accuracy in which the underlying state (or, target trajectory) can be estimated using only single sensor data. Next, we use these likelihoods as edge potentials of a pairwise Markov Random Field to model the global problem. The resulting estimation algorithm consists of consecutive separable likelihood evaluations and belief propagation message passings. We demonstrate this strategy in a simulation example.