Documents
Poster
DNN-BASED SPEAKER-ADAPTIVE POSTFILTERING WITH LIMITED ADAPTATION DATA FOR STATISTICAL SPEECH SYNTHESIS SYSTEMS
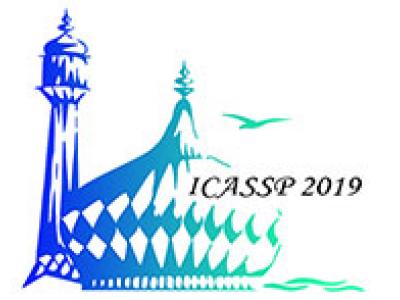
- Citation Author(s):
- Submitted by:
- Mirac Ozturk
- Last updated:
- 15 February 2019 - 5:17am
- Document Type:
- Poster
- Document Year:
- 2019
- Event:
- Presenters:
- Miraç Göksu Öztürk
- Paper Code:
- 2841
- Categories:
- Log in to post comments
Deep neural networks (DNNs) have been successfully deployed for acoustic modelling in statistical parametric speech synthesis (SPSS) systems. Moreover, DNN-based postfilters (PF) have also been shown to outperform conventional postfilters that are widely used in SPSS systems for increasing the quality of synthesized speech. However, existing DNN-based postfilters are trained with speaker-dependent databases. Given that SPSS systems can rapidly adapt to new speakers from generic models, there is a need for DNN-based postfilters that can adapt to new speakers with minimal adaptation data. Here, we compare DNN-, RNN-, and CNN-based postfilters together with adversarial (GAN) training and cluster-based initialization (CI) for rapid adaptation. Results indicate that the feedforward (FF) DNN, together with GAN and CI, significantly outperforms the other recently proposed postfilters.