Documents
Presentation Slides
DNN-Based Speech Presence Probability Estimation for Multi-Frame Single-Microphone Speech Enhancement
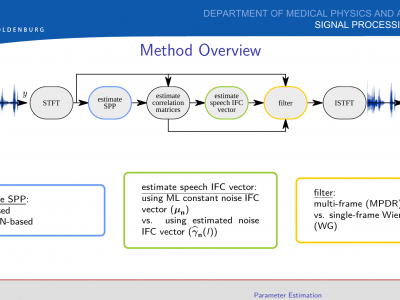
- Citation Author(s):
- Submitted by:
- Marvin Tammen
- Last updated:
- 15 May 2020 - 6:12am
- Document Type:
- Presentation Slides
- Document Year:
- 2020
- Event:
- Presenters:
- Marvin Tammen
- Paper Code:
- AUD-L7.3
- Categories:
- Log in to post comments
Multi-frame approaches for single-microphone speech enhancement, e.g., the multi-frame minimum-power-distortionless-response (MFMPDR) filter, are able to exploit speech correlations across neighboring time frames. In contrast to single-frame approaches such as the Wiener gain, it has been shown that multi-frame approaches achieve a substantial noise reduction with hardly any speech distortion, provided that an accurate estimate of the correlation matrices and especially the speech interframe correlation (IFC) vector is available. Typical estimation procedures of the IFC vector require an estimate of the speech presence probability (SPP) in each time-frequency (TF) bin. In this paper, we propose to use a bi-directional long short-term memory deep neural network (DNN) to estimate the SPP for each TF bin. Aiming at achieving a robust performance, the DNN is trained for various noise types and within a large signal-to-noise-ratio range. Experimental results show that the MFMPDR in combination with the proposed datadriven SPP estimator yields an increased speech quality compared to a state-of-the-art model-based SPP estimator. Furthermore, it is confirmed that exploiting interframe correlations in the MFMPDR is beneficial when compared to the Wiener gain especially in adverse scenarios.