Documents
Poster
DPCCN: Densely-Connected Pyramid Complex Convolutional Network for Robust Speech Separation And Extraction
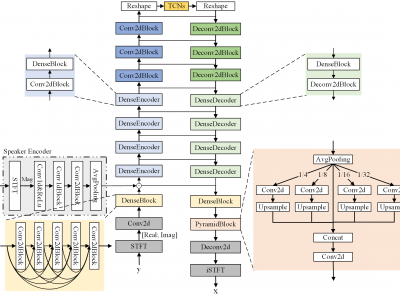
- Citation Author(s):
- Submitted by:
- jy han
- Last updated:
- 4 May 2022 - 10:29pm
- Document Type:
- Poster
- Event:
- Presenters:
- Jiangyu Han
- Paper Code:
- 1390
- Categories:
- Log in to post comments
In recent years, a number of time-domain speech separation methods have been proposed. However, most of them are very sensitive to the environments and wide domain coverage tasks. In this paper, from the time-frequency domain perspective, we propose a densely-connected pyramid complex convolutional network, termed DPCCN, to improve the robustness of speech separation under complicated conditions. Furthermore, we generalize the DPCCN to target speech extraction (TSE) by integrating a new specially designed speaker encoder. Moreover, we also investigate the robustness of DPCCN to unsupervised cross-domain TSE tasks. A Mixture-Remix approach is proposed to adapt the target domain acoustic characteristics for fine-tuning the source model. We evaluate the proposed methods not only under noisy and reverberant in-domain condition, but also in clean but cross-domain conditions. Results show that for both speech separation and extraction, the DPCCN-based systems achieve significantly better performance and robustness than the currently dominating time-domain methods, especially for the crossdomain tasks. Particularly, we find that the Mixture-Remix finetuning with DPCCN significantly outperforms the TD-SpeakerBeam for unsupervised cross-domain TSE, with around 3.5 dB SISNR improvement on target domain test set, without any source domain performance degradation.