Documents
Poster
DROPFL: Client Dropout Attacks Against Federated Learning Under Communication Constraints
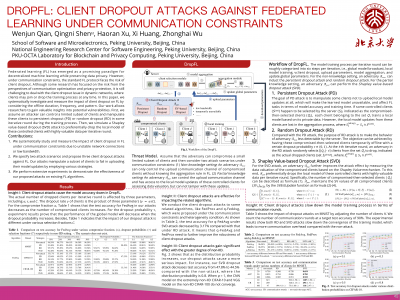
- DOI:
- 10.60864/tf1s-va59
- Citation Author(s):
- Submitted by:
- Wenjun Qian
- Last updated:
- 6 June 2024 - 10:50am
- Document Type:
- Poster
- Document Year:
- 2024
- Event:
- Presenters:
- Wenjun Qian
- Categories:
- Log in to post comments
Federated learning (FL) has emerged as a promising paradigm for decentralized machine learning while preserving data privacy. However, under communication constraints, the standard FL protocol faces the risk of client dropout. Although some research has focused on the risk from the perspectives of communication optimization and privacy protection, it is still challenging to deal with the client dropout issue in dynamic networks, where clients may join or drop the training process at any time. In this paper, we systematically investigate and measure the impact of client dropout on FL by considering the offline duration, frequency, and pattern. Our work allows researchers to gain valuable insights into potential vulnerabilities. First, we assume an attacker can control a limited subset of clients and manipulate these clients to persistent dropout (PD) or random dropout (RD) in some iterative round during the training process. Then, we simulate a Shapley value-based dropout (SVD) attack to preferentially drop the local model of these controlled clients with highly valuable data per iterative round. Furthermore, extensive experiments are performed to demonstrate the effectiveness of our proposed attacks on existing FL algorithms.