Documents
Poster
Poster
Dropout approaches for LSTM based speech recognition systems
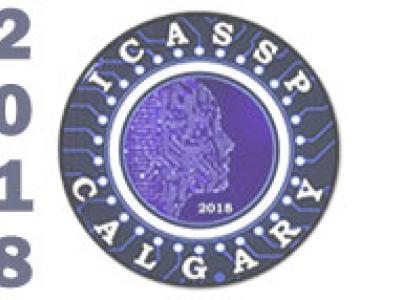
- Citation Author(s):
- Submitted by:
- Jayadev Billa
- Last updated:
- 19 April 2018 - 2:52pm
- Document Type:
- Poster
- Document Year:
- 2018
- Event:
- Presenters:
- Jayadev Billa
- Paper Code:
- SP-P18.8
- Categories:
- Log in to post comments
In this paper we examine dropout approaches in a Long Short Term Memory (LSTM) based automatic speech recognition (ASR) system trained with the Connectionist Temporal Classification (CTC) loss function. In particular, using an Eesen based LSTM-CTC speech recognition system, we present dropout implementations that result in significant improvements in speech recognizer performance on Librispeech and GALE Arabic datasets, with 24.64% and 13.75% relative reduction in word error rates (WER) from their respective baselines.