Documents
Presentation Slides
A dual attention dilated residual network for liver lesion classification and localization on CT images
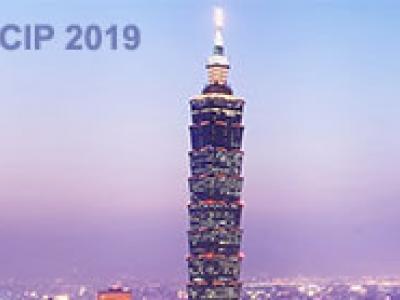
- Citation Author(s):
- Submitted by:
- Xiao Chen
- Last updated:
- 16 September 2019 - 1:10am
- Document Type:
- Presentation Slides
- Document Year:
- 2019
- Event:
- Presenters:
- Xiao Chen
- Paper Code:
- 2034
- Categories:
- Log in to post comments
Automatic liver lesion classification on computed
tomography images is of great importance to early cancer
diagnosis and remains a challenging task. State-of-the-art
liver lesion classification algorithms are currently based on
manually selected regions of interest (ROIs) or automatically
detected ROIs. However, liver lesions usually vary in size
and shape, which makes the ROI selection process laborintensive
and also poses an obstacle to automatic lesion
detection. In this paper, we propose a dual-attention dilated
residual network (DADRN) as a potential solution to lesion
classification task without manual ROI selection or automatic
lesion detection. We incorporated a novel dual-attention
module in order to capture the non-local feature dependencies
and help the deep neural network focus on the lesion area by
enlarging the difference between the lesion area and nonlesion
area. To the best of our knowledge, we are the first to
employ the self-attention mechanism to address liver lesion
classification task. In addition, the well-trained DADRN can
be used for weakly-supervised lesion localization without any
architectural change or retraining. Experiment results show
that DADRN could achieve a lesion classification accuracy
comparable to that of the state-of-the-art ROI-based method
and outperformed state-of-the-art attention-based approaches
in both liver lesion classification and localization tasks.