Documents
Poster
Dynamic ASR pathways: An Adaptive Masking Approach Towards Efficient Pruning of a Multilingual ASR Model
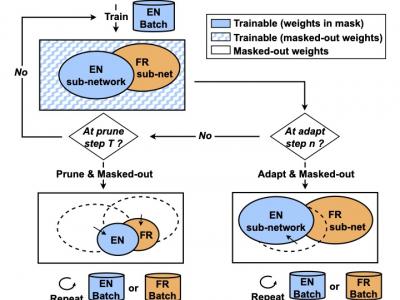
- DOI:
- 10.60864/n3ad-6065
- Citation Author(s):
- Submitted by:
- Jiamin Xie
- Last updated:
- 6 June 2024 - 10:32am
- Document Type:
- Poster
- Document Year:
- 2024
- Event:
- Presenters:
- Andros Tjandra
- Paper Code:
- SLP-P21.10
- Categories:
- Log in to post comments
Neural network pruning offers an effective method for compressing a multilingual automatic speech recognition (ASR) model with minimal performance loss. However, it entails several rounds of pruning and re-training needed to be run for each language. In this work, we propose the use of an adaptive masking approach in two scenarios for pruning a multilingual ASR model efficiently, each resulting in sparse monolingual models or a sparse multilingual model (named as Dynamic ASR Pathways). Our approach dynamically adapts the sub-network, avoiding premature decisions about a fixed sub-network structure. We show that our approach outperforms existing pruning methods when targeting sparse monolingual models. Further, we illustrate that Dynamic ASR Pathways jointly discovers and trains better sub-networks (pathways) of a single multilingual model by adapting from different sub-network initializations, thereby reducing the need for language-specific pruning.