Documents
Presentation Slides
DYNAMIC MULTI-RATER GAUSSIAN MIXTURE REGRESSION INCORPORATING TEMPORAL DEPENDENCIES OF EMOTION UNCERTAINTY USING KALMAN FILTERS
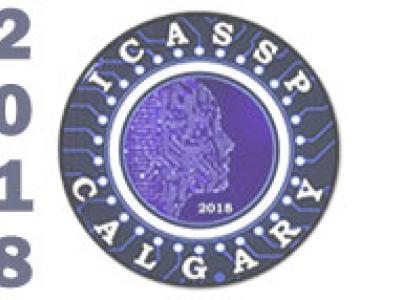
- Citation Author(s):
- Submitted by:
- Ting Dang
- Last updated:
- 23 April 2018 - 6:03pm
- Document Type:
- Presentation Slides
- Document Year:
- 2018
- Event:
- Presenters:
- Ting Dang
- Paper Code:
- 4062
- Categories:
- Keywords:
- Log in to post comments
Predicting continuous emotion in terms of affective attrib-utes has mainly been focused on hard labels, which ignored the ambiguity of recognizing certain emotions. This ambigu-ity may result in high inter-rater variability and in turn caus-es varying prediction uncertainty with time. Based on the assumption that temporal dependencies occur in the evolu-tion of emotion uncertainty, this paper proposes a dynamic multi-rater Gaussian Mixture Regression (GMR), aiming to obtain the emotion uncertainty prediction reflected by multi-raters by taking into account their temporal dependencies. This framework is achieved by incorporating feedforward and backward Kalman filters into GMR to estimate the time-dependent label distribution that reflects the emotion uncertainty. It also provides the benefits of relaxing the label distribution of Gaussian assumption to that of a Gaussian Mixture Model (GMM). In addition, a new measurement to estimate emotion uncertainty from GMM as the local variability is adopted. Experiments conducted on the RECOLA database reveal that incorporating temporal dependencies is critical for emotion uncertainty prediction with 17% relative improvement for arousal, and that the proposed framework for emotion uncertainty prediction shows potential in conventional emotion attribute prediction.