Documents
Research Manuscript
ENACT: Entropy-based Clustering of Attention Input for Reducing the Computational Resources of Object Detection Transformers - Supplementary Material
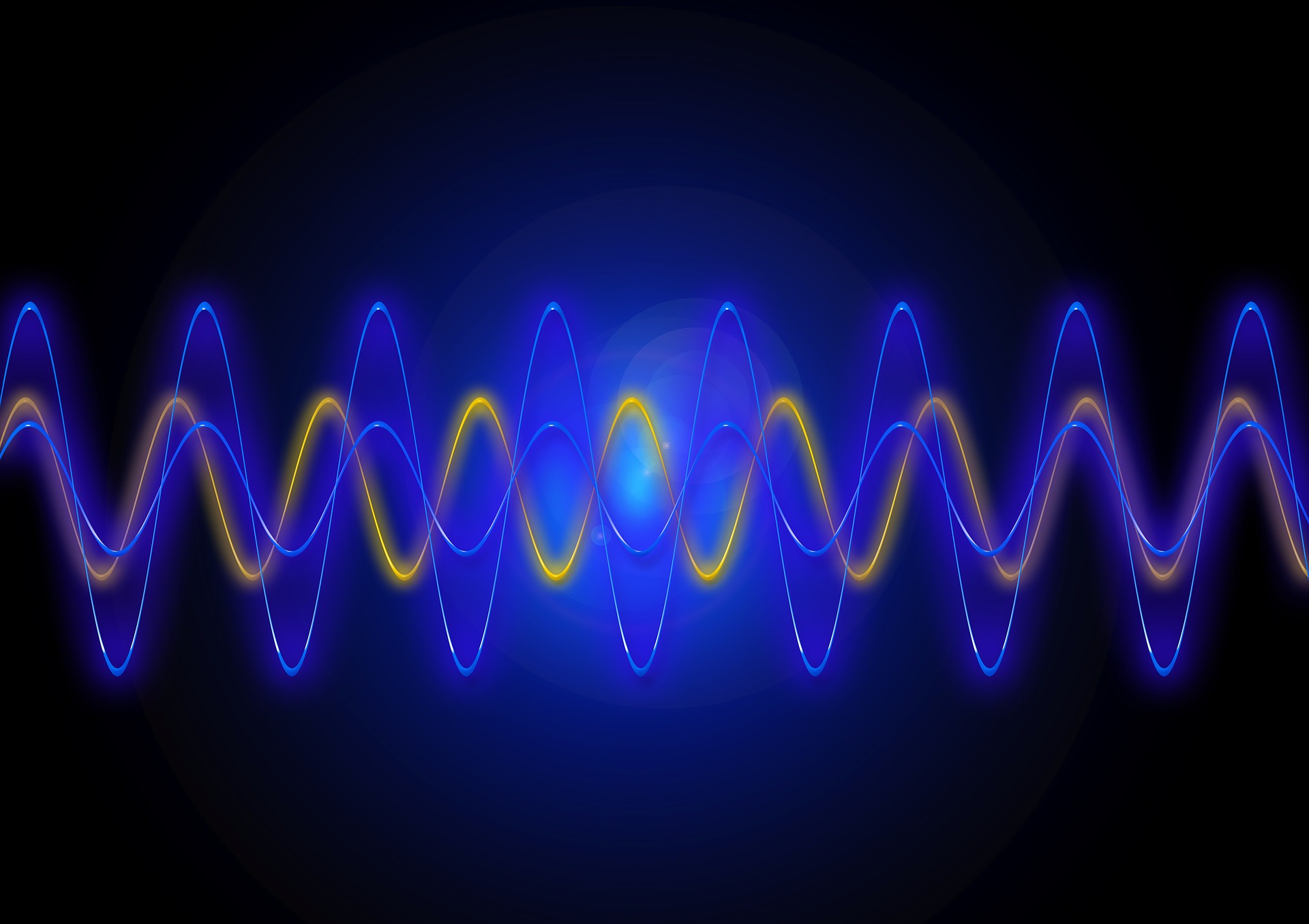
- DOI:
- 10.60864/9s39-4e17
- Citation Author(s):
- Submitted by:
- GEORGIOS SAVATHRAKIS
- Last updated:
- 30 January 2025 - 7:11am
- Document Type:
- Research Manuscript
- Categories:
- Keywords:
- Log in to post comments
Transformers demonstrate competitive performance in terms of precision on the problem of vision-based object detection. However, they require considerable computational resources due to the quadratic size of the attention weights.
In this work, we propose to cluster the transformer input on the basis of its entropy, due to its similarity between same object pixels. This is expected to reduce GPU usage during training, while maintaining reasonable accuracy. This idea is realized with an implemented module that is called ENACT, which serves as a plug-in to any multi-head self-attention based transformer network. Experiments on the COCO object detection dataset and three detection transformers demonstrate that the requirements on memory are reduced, while the detection accuracy is degraded only slightly.