Documents
Presentation Slides
END-TO-END ASR-ENHANCED NEURAL NETWORK FOR ALZHEIMER’S DISEASE DIAGNOSIS
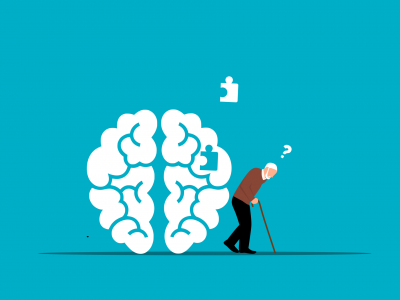
- Citation Author(s):
- Submitted by:
- Jiancheng Gui
- Last updated:
- 6 May 2022 - 9:57pm
- Document Type:
- Presentation Slides
- Event:
- Presenters:
- Jiancheng Gui
- Paper Code:
- SPE-88.5
- Categories:
- Log in to post comments
This paper presents an approach to Alzheimer’s disease (AD) diagnosis from spontaneous speech using an end-to-end ASR-enhanced neural network. Under the condition that only audio data are provided and accurate transcripts are unavailable, this paper proposes a system that can analyze utterances to differentiate between AD patients, healthy controls, and individuals with mild cognitive impairment. The ASR-enhanced model comprises automatic speech recognition (ASR) with an encoder-decoder structure and the encoder followed by an AD classification network. The encoder takes a Mel spectrogram as input and transforms it into high-level acoustic features that correlate with AD. The classification network then maps intermediate acoustic features to three categories. In the training phase, the AD classification and speech recognition tasks are optimized simultaneously. Experimental results obtained from an AD recognition dataset of Chinese spontaneous speech illustrate the effectiveness of integrating ASR into AD diagnosis in an end-to-end manner. Further, our model has low dependency on accurate ASR transcripts. This work achieved accuracy scores of 89.1% and 82.6% for long and short utterance tracks, respectively.