Documents
Poster
END-TO-END HIERARCHICAL LANGUAGE IDENTIFICATION SYSTEM
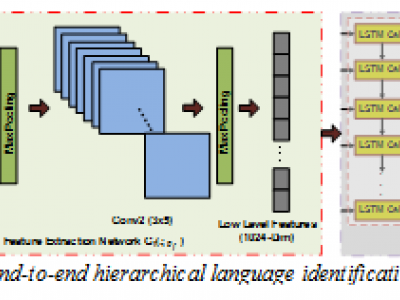
- Citation Author(s):
- Submitted by:
- Saad Irtza
- Last updated:
- 12 April 2018 - 9:20pm
- Document Type:
- Poster
- Document Year:
- 2018
- Event:
- Presenters:
- Ting Dang
- Paper Code:
- SP-P4
- Categories:
- Log in to post comments
Recently, hierarchical language identification systems have shown significant improvement over single level systems in both closed and open set language identification tasks. However, developing such a system requires the features and classifier selection at each node in the hierarchical structure to be hand crafted. Motivated by the superior ability of end-to-end deep neural network architecture to jointly optimize the feature extraction and classification process, we propose a novel approach developing an end-to-end hierarchical language identification system. The proposed approach also demonstrates the in-built ability of the end-to-end hierarchical structure training that enables an out-of-set language model, without using any additional out-of-set language training data. Experiments are conducted on the NIST LRE 2015 data set. The overall results show relative improvements of 18.6% and 27.3% in terms of Cavg in closed and open set tasks over the corresponding baseline systems.