Documents
Poster
END-TO-END LANGUAGE RECOGNITION USING ATTENTION BASED HIERARCHICAL GATED RECURRENT UNIT MODELS
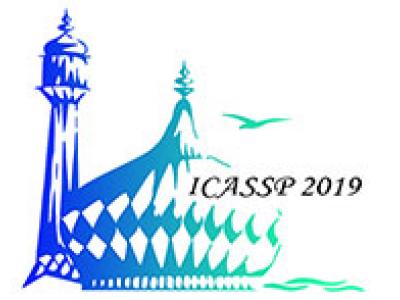
- Citation Author(s):
- Submitted by:
- Bharat padi
- Last updated:
- 10 May 2019 - 2:30am
- Document Type:
- Poster
- Document Year:
- 2019
- Event:
- Presenters:
- Bharat Kumar Padi
- Paper Code:
- SLP-P1.4
- Categories:
- Log in to post comments
The task of automatic language identification (LID) involving multiple dialects of the same language family on short speech recordings is a challenging problem. This can be further complicated for short-duration audio snippets in the presence of noise sources. In these scenarios, the identity of the language/dialect may be reliably present only in parts of the speech embedded in the temporal sequence. The conventional approaches to LID (and for speaker recognition) ignore the sequence information by extracting long-term statistical summary of the recording assuming independence of the feature frames. In this paper, we propose to develop an end-to-end neural network framework utilizing short-sequence information in language recognition. A hierarchical gated recurrent unit (HGRU) model with attention module is proposed for incorporating relevance in language recognition, where parts of speech data are weighted more based on their relevance for the language recognition task. Experiments are performed using the language recognition task in NIST LRE 2017 Challenge using clean, noisy and multi-speaker speech data. In these experiments, the proposed approach yields significant improvements over the conventional i-vector based language recognition approaches as well as a previously proposed approach to language recognition using recurrent networks.