Documents
Presentation Slides
END-TO-END SPEECH RECOGNITION CONTEXTUALIZATION WITH LARGE LANGUAGE MODELS
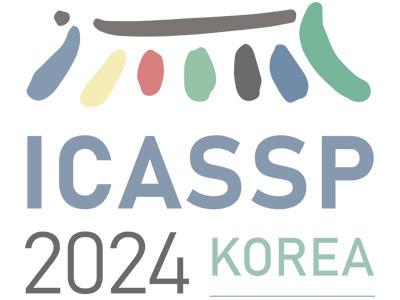
- Citation Author(s):
- Submitted by:
- Egor Lakomkin
- Last updated:
- 16 April 2024 - 2:49am
- Document Type:
- Presentation Slides
- Document Year:
- 2024
- Event:
- Presenters:
- EGOR LAKOMKIN
- Paper Code:
- SLP-L5.6
- Categories:
- Log in to post comments
In recent years, Large Language Models (LLMs) have garnered significant attention from the research community due to their exceptional performance and generalization capabilities. In this paper, we introduce a novel method for contextualizing speech recognition models incorporating LLMs. Our approach casts speech recognition as a mixed-modal language modeling task based on a pretrained LLM. We provide audio features, along with optional text tokens for context, to train the system to complete transcriptions in a decoderonly fashion. As a result, the system is implicitly incentivized to learn how to leverage unstructured contextual information during training. Our empirical results demonstrate a significant improvement in performance, with a 6% WER reduction when additional textual context is provided. Moreover, we find that our method performs competitively and improve by 7.5% WER overall and 17% WER on rare words against a baseline contextualized RNN-T system that has been trained on more than twenty five times larger speech dataset. Overall, we demonstrate that by only adding a handful number of trainable parameters via adapters, we can unlock contextualized speech recognition capability for the pretrained LLM while keeping the same text-only input functionality