Documents
Poster
Enhanced Recurrent Neural Network for Combining Static and Dynamic Features for Credit Card Default Prediction
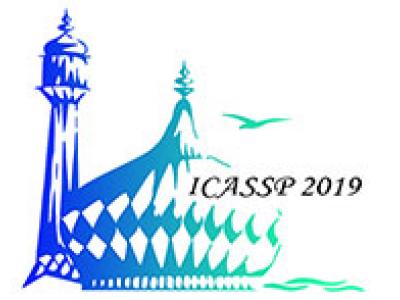
- Citation Author(s):
- Submitted by:
- Te-Cheng Hsu
- Last updated:
- 8 May 2019 - 9:27am
- Document Type:
- Poster
- Document Year:
- 2019
- Event:
- Presenters:
- Te-Cheng Hsu
- Paper Code:
- DISPS-P3
- Categories:
- Log in to post comments
Deep learning models have been shown to be capable of extracting high-level representations from the increasing amount of customer-level data generated via fast-growing financial activities. In financial data, dynamic features that evolve with time are commonly observed. However, such time dependencies are often ignored in classical classification models. In this study, we propose to learn a Recurrent Neural Network (RNN) feature extractor with GRU on credit card payment history to leverage the time dependencies embedded in these dynamic features. Input sequences are first preprocessed by this feature extractor. The extracted dynamic features along with the static features are then utilized to train an enhanced RNN model (RNN-RF) to predict credit card client defaults. Numerical experiments confirmed that the enhanced RNN predictor indeed provides the best performance in both lift index (0.659) and AUC (0.782) compared to the other benchmark models. The proposed model allows us to effectively combine static and dynamic features to provide superior predictive performance for financial data.