Documents
Presentation Slides
Enhancing Adversarial Transferability in Object Detection with Bidirectional Feature Distortion
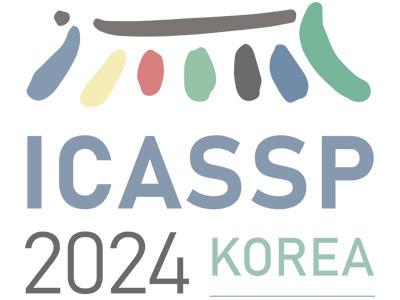
- DOI:
- 10.60864/prhn-q635
- Citation Author(s):
- Submitted by:
- XINLONG DING
- Last updated:
- 6 June 2024 - 10:32am
- Document Type:
- Presentation Slides
- Document Year:
- 2024
- Event:
- Presenters:
- DING XINLONG
- Categories:
- Log in to post comments
Previous works have shown that perturbing internal-layer features can significantly enhance the transferability of black-box attacks in classifiers. However, these methods have not achieved satisfactory performance when applied to detectors due to the inherent differences in features between detectors and classifiers. In this paper, we introduce a concise and practical untargeted adversarial attack in a label-free manner, which leverages only the feature extracted from the backbone model. By implicitly suppressing the critical feature elements for detection while enhancing the candidate object-relevant elements corresponding to possible detection boxes, we conduct a Bidirectional Feature Distortion Attack (BFDA). Experimental results show that BFDA achieves state-of-the-art black-box transferability on various detector architectures.