Documents
Poster
EVALUATING MODELS OF DYNAMIC FUNCTIONAL CONNECTIVITY USING PREDICTIVE CLASSIFICATION ACCURACY
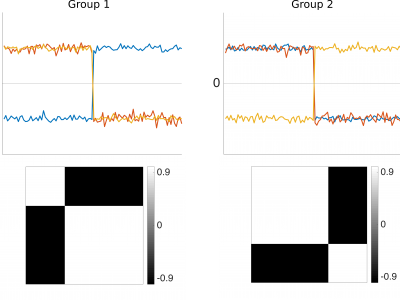
- Citation Author(s):
- Submitted by:
- Soeren Nielsen
- Last updated:
- 13 April 2018 - 10:02am
- Document Type:
- Poster
- Document Year:
- 2018
- Event:
- Presenters:
- Soeren FV Nielsen
- Paper Code:
- MLSP-P4.7
- Categories:
- Log in to post comments
Dynamic functional connectivity has become a prominent approach for tracking the changes of macroscale statistical dependencies between regions in the brain. Effective parametrization of these statistical dependencies, referred to as brain states, is however still an open problem. We investigate different emission models in the hidden Markov model framework, each representing certain assumptions about dynamic changes in the brain. We evaluate each model by how well they can discriminate between schizophrenic patients and healthy controls based on a group independent component analysis of resting-state functional magnetic resonance imaging data. We find that simple emission models without full covariance matrices can achieve similar classification results as the models with more parameters. This raises questions about the predictability of dynamic functional connectivity in comparison to simpler dynamic features when used as biomarkers. However, we must stress that there is a distinction between characterization and classification, which has to be investigated further.