Documents
Poster
Exact Recovery by Semidefinite Programming in the Binary Stochastic Block Model with Partially Revealed Side Information
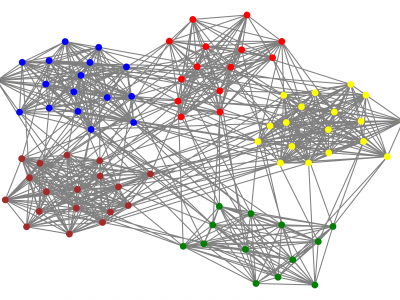
- Citation Author(s):
- Submitted by:
- Mohammad Esmaeili
- Last updated:
- 9 May 2019 - 9:07pm
- Document Type:
- Poster
- Document Year:
- 2019
- Event:
- Presenters:
- Aria Nosratinia
- Paper Code:
- 3846
- Categories:
- Log in to post comments
Semidefinite programming has been shown to be both efficient and asymptotically optimal in solving community detection problems, as long as observations are purely graphical in nature. In this paper, we extend this result to observations that have both a graphical and a non-graphical component. We consider the binary censored block model with $n$ nodes and study the effect of partially revealed labels on the performance of semidefinite programming. Essentially, we ask the question: do partially revealed labels help the semidefinite programming solution as much as they help the maximum likelihood solution? Our results are two fold. First, we show that partially revealed labels change the phase transition of exact recovery if and only if they grow no slower than $\Omega(\log(n))$. Second, we show that the semidefinite programming relaxation of maximum likelihood can achieve exact recovery down to the optimal threshold under partially revealed labels.