Documents
Presentation Slides
Explainable Fact-Checking Through Question Answering
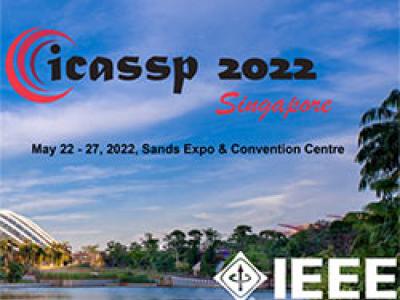
- Citation Author(s):
- Submitted by:
- Jing Yang
- Last updated:
- 4 May 2022 - 8:50pm
- Document Type:
- Presentation Slides
- Document Year:
- 2022
- Event:
- Presenters:
- Jing Yang
- Paper Code:
- SS-13.2
- Categories:
- Log in to post comments
Misleading or false information has been creating chaos in some places around the world. To mitigate this issue, many researchers have proposed automated fact-checking methods to fight the spread of fake news. However, most methods cannot explain the reasoning behind their decisions, failing to build trust between machines and humans using such technology. Trust is essential for fact-checking to be applied in the real world. Here, we address fact-checking explainability through question answering. In particular, we propose generating questions and answers from claims and answering the same questions from evidence. We also propose an answer comparison model with an attention mechanism attached to each question. Leveraging question answering as a proxy, we break down automated fact-checking into several steps — this separation aids models’ explainability as it allows for more detailed analysis of their decision-making processes. Experimental results show that the proposed model can achieve state-of-the-art performance while providing reasonable explainable capabilities.