Documents
Poster
Exploiting noisy web data by OOV ranking for low-resource keyword search
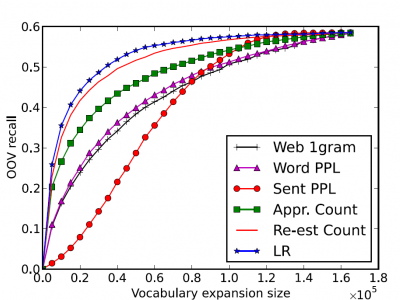
- Citation Author(s):
- Submitted by:
- Zhipeng Chen
- Last updated:
- 15 October 2016 - 7:55am
- Document Type:
- Poster
- Document Year:
- 2016
- Event:
- Presenters:
- Zhipeng Chen
- Paper Code:
- P3-11
- Categories:
- Log in to post comments
Spoken keyword search in low-resource condition suffers from out-of-vocabulary (OOV) problem and insufficient text data for language model (LM) training. Web-crawled text data is used to expand vocabulary and to augment language model. However, the mismatching between web text and the target speech data brings difficulties to effective utilization. New words from web data need an evaluation to exclude noisy words or introduce proper probabilities. In this paper, several criteria to rank new words from web data are investigated and are used as features
for logistic regression. In the IV keyword case, top N words are selected to expand the vocabulary. In the OOV keyword case, all words are used for expansion but unigram probabilities are re-assigned by Zipf’s law. On Swahili keyword search, after further text filtering and LM interpolation, this strategy is observed to outperform a strong and commonly used baseline method for data selection.