Documents
Poster
Poster
Face Recognition with Disentangled Facial Representation Learning and Data Augmentation
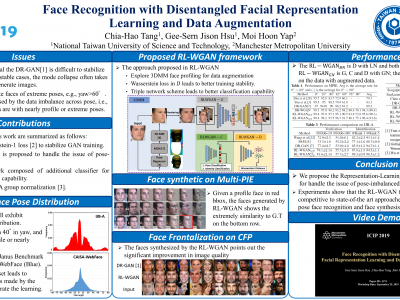
- Citation Author(s):
- Submitted by:
- Chia-Hao Tang
- Last updated:
- 19 September 2019 - 6:16am
- Document Type:
- Poster
- Document Year:
- 2019
- Event:
- Presenters:
- Chia-Hao Tang
- Paper Code:
- 3371
- Categories:
- Log in to post comments
We address two issues for tackling face recognition across pose, one is disentangled representation learning and the other is training data augmentation. To have better training properties, we propose the Representation-Learning Wasserstein-GAN (RL-WGAN) with three component networks for learning the disentangled facial representation. As the learning based on imbalanced data often leads to biased estimation, we proposed a data augmentation scheme that exploits the 3D Morphable Model (3DMM) for generating faces of desired poses. The RL-WGAN and the data augmentation are verified in the experiments with benchmark databases, and compared with contemporary approaches for performance evaluation.