Documents
Presentation Slides
FAST BLOCK-SPARSE ESTIMATION FOR VECTOR NETWORKS
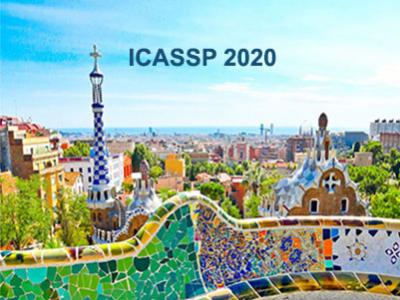
- Citation Author(s):
- Submitted by:
- Zuogong Yue
- Last updated:
- 15 May 2020 - 3:00am
- Document Type:
- Presentation Slides
- Document Year:
- 2020
- Event:
- Presenters:
- Zuogong Yue
- Paper Code:
- 1151
- Categories:
- Log in to post comments
While there is now a significant literature on sparse inverse covariance estimation, all that literature, with only a couple of exceptions, has dealt only with univariate (or scalar) net- works where each node carries a univariate signal. However in many, perhaps most, applications, each node may carry multivariate signals representing multi-attribute data, possibly of different dimensions. Modelling such multivariate (or vector) networks requires fitting block-sparse inverse covariance matrices. Here we achieve maximal block sparsity by maximizing a block-l0-sparse penalized likelihood. There is only one previous algorithm that already does this, but it does not scale. Here we address key computational bottlenecks and develop a new algorithm which is much faster and has massively reduced requirements on matrix conditioning. A benchmark study shows a computational speed-up by many orders of magnitude.
blockSpGGM.pdf
