Documents
Poster
A feature fusion method based on extreme learning machine for speech emotion recognition
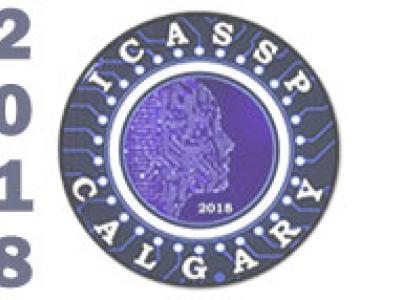
- Citation Author(s):
- Submitted by:
- Lili Guo
- Last updated:
- 12 April 2018 - 12:07pm
- Document Type:
- Poster
- Document Year:
- 2018
- Event:
- Paper Code:
- MLSP-P6.7
- Categories:
- Log in to post comments
Speech emotion recognition is important to understand users' intention in human-computer interaction. However, it is a challenging task partly because we cannot clearly know which feature and model are effective to distinguish emotions. Previous studies utilize convolutional neural network (CNN) directly on spectrograms to extract features, and bidirectional long short term memory (BLSTM) is the state-of-the-art model. However, there are two problems of CNN-BLSTM. Firstly, it doesn't utilize heuristic features based on priori knowledge. Secondly, BLSTM has a complex structure and high complexity in training. To address the first problem, we propose a feature fusion method that combines CNN-based features and heuristic-based discriminative features which are extracted from heuristic features using deep neural network (DNN). In addition, we utilize extreme learning machine (ELM) instead of BLSTM to solve the second problem. The experiments conducted on EmoDB and our method leads to 40% relative error reduction in F1-score compared to CNN-BLSTM.