Documents
Presentation Slides
Feature LMS Algorithms
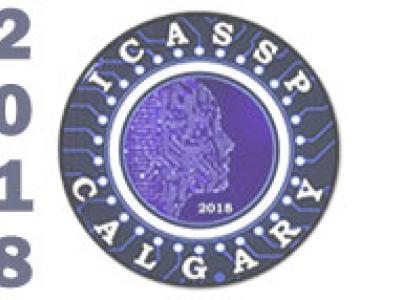
- Citation Author(s):
- Submitted by:
- Paulo Diniz
- Last updated:
- 13 April 2018 - 11:20pm
- Document Type:
- Presentation Slides
- Document Year:
- 2018
- Event:
- Presenters:
- Paulo S. R. Diniz
- Paper Code:
- ICASSP18001
- Categories:
- Log in to post comments
In recent years, there is a growing effort in the learning algorithms
area to propose new strategies to detect and exploit
sparsity in the model parameters. In many situations, the
sparsity is hidden in the relations among these coefficients
so that some suitable tools are required to reveal the potential
sparsity. This work proposes a set of LMS-type algorithms,
collectively called Feature LMS (F-LMS) algorithms, setting
forth a hidden feature of the unknown parameters, which ultimately
would improve convergence speed and steady-state
mean-squared error. The key idea is to apply linear transformations,
by means of the so-called feature matrices, to reveal
the sparsity hidden in the coefficient vector, followed by a
sparsity-promoting penalty function to exploit such sparsity.
Some F-LMS algorithms for lowpass and highpass systems
are also introduced by using simple feature matrices that require
only trivial operations. Simulation results demonstrate
that the proposed F-LMS algorithms bring about several performance
improvements whenever the hidden sparsity of the
parameters is exposed.