Documents
Poster
FreeTalker: Controllable Speech and Text-Driven Gesture Generation Based on Diffusion Models for Enhanced Speaker Naturalness
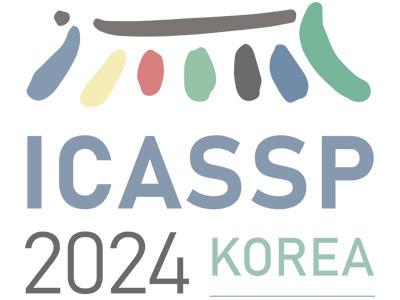
- DOI:
- 10.60864/d7q6-7f88
- Citation Author(s):
- Submitted by:
- SiCheng Yang
- Last updated:
- 8 April 2024 - 5:46am
- Document Type:
- Poster
- Document Year:
- 2024
- Event:
- Presenters:
- Sicheng Yang
- Paper Code:
- MMSP-P6.1
- Categories:
- Keywords:
- Log in to post comments
Current talking avatars mostly generate co-speech gestures based on audio and text of the utterance, without considering the non-speaking motion of the speaker. Furthermore, previous works on co-speech gesture generation have designed network structures based on individual gesture datasets, which results in limited data volume, compromised generalizability, and restricted speaker movements. To tackle these issues, we introduce FreeTalker, which, to the best of our knowledge, is the first framework for the generation of both spontaneous (e.g., co-speech gesture) and non-spontaneous (e.g., moving around the podium) speaker motions. Specifically, we train a diffusion-based model for speaker motion generation that employs unified representations of both speech-driven gestures and text-driven motions, utilizing heterogeneous data sourced from various motion datasets. During inference, we utilize classifier-free guidance to highly control the style in the clips. Additionally, to create smooth transitions between clips, we utilize DoubleTake, a method that leverages a generative prior and ensures seamless motion blending. Extensive experiments show that our method generates natural and controllable speaker movements. Our code, model, and demo are are available at project page.
Comments
First Upload
First Upload