Documents
Presentation Slides
Fully Integerized End-to-End Learned Image Compression
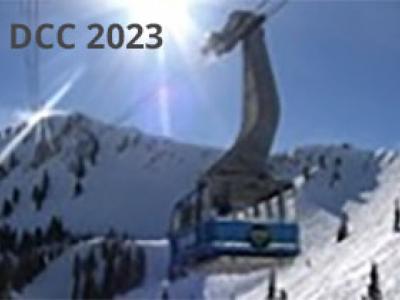
- Citation Author(s):
- Submitted by:
- Yimian Fang
- Last updated:
- 28 February 2023 - 7:28am
- Document Type:
- Presentation Slides
- Document Year:
- 2023
- Event:
- Presenters:
- Yimian Fang
- Paper Code:
- DCC-178
- Categories:
- Keywords:
- Log in to post comments
End-to-end learned image compression (LIC) has become promising alternatives for lossy image compression. However, deployments of LIC models are restricted, due to excessive network parameters and high computational complexity. Existing LIC models realized throughout with integer networks are significantly degraded in rate-distortion (R-D) performance. In this paper, we propose a novel fully integerized model for LIC that leverages channel-wise weight and activation quantization. To alleviate R-D performance loss caused by quantization, we develop an internal bit width increment with nonlinear logarithmic mapping for integer-based convolution operations, and apply outlier channel splitting to realize the INT8 model. Moreover, activation equalization is utilized to balance the channel-wise distribution of activations to facilitate network inference for compression. The proposed model is the first to achieve negligible loss in R-D performance in support of integer-only arithmetic for LIC. Experimental results show that the proposed fully integerized model achieves a reduction of 75% storage cost with subtle performance degradation compared to full-precision pre-trained models, and outperforms existing integer-only networks.