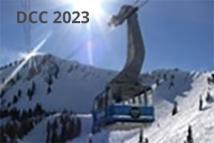
DCC 2023 Conference - The Data Compression Conference (DCC) is an international forum for current work on data compression and related applications. Both theoretical and experimental work are of interest. Visit the DCC 2023 website.
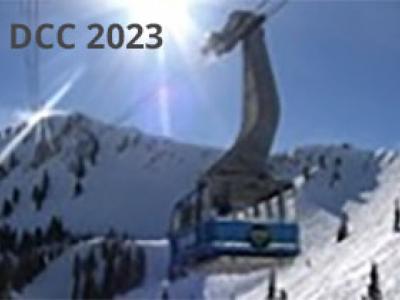
- Categories:
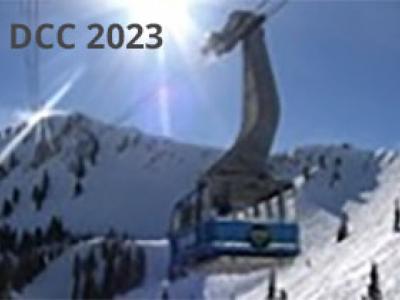
- Read more about Constrained Autoencoders: Incorporating equality constraints in learned scientific data compression
- Log in to post comments
As today's scientific simulations with high-performance computing produce an extremely large amount of data, reliable data compression techniques are becoming important. In scientific applications, downstream quantities derived from the original or primary data (PD) are crucial for post-analysis, and therefore these Quantities of Interest (QoI) are required to be preserved during compression. While autoencoders (AEs) have recently been used for the compression of scientific datasets, there is a clear lack in the literature w.r.t.
- Categories:
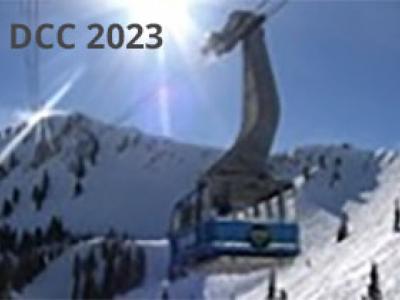
- Read more about Adaptive and Scalable Compression of Multispectral Images using VVC
- Log in to post comments
The VVC codec is applied to the task of multispectral image (MSI) compression using adap- tive and scalable coding structures. In a “plain” VVC approach, concepts from picture-to- picture temporal prediction are employed for decorrelation along the MSI’s spectral dimen- sion. The popular principle component analysis (PCA) for spectral decorrelation is further evaluated in combination with VVC intra-coding for spatial decorrelation. This approach is referred to as PCA-VVC.
- Categories:
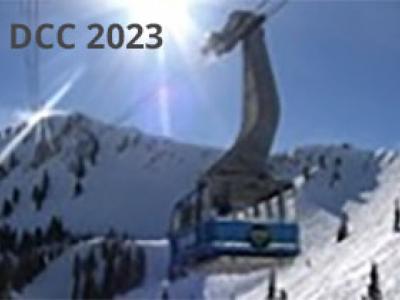
- Read more about Semantically Adaptive JND Modeling with Object-wise Feature Characterization and Cross-object Interaction
- Log in to post comments
- Categories:
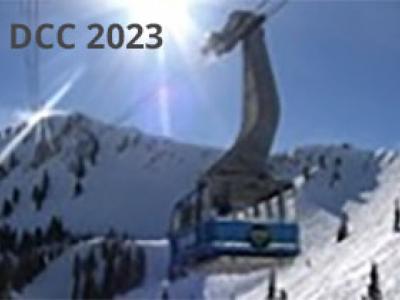
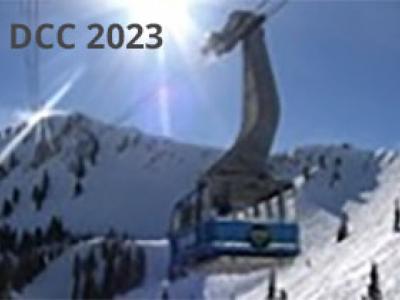
- Read more about Rate-Distortion via Energy-Based Models
- Log in to post comments
- Categories:
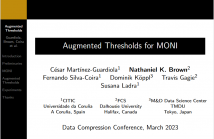
- Read more about Augmented Thresholds for MONI
- Log in to post comments
MONI (Rossi et al., 2022) can store a pangenomic dataset T in small space and later, given a pattern P, quickly find the maximal exact matches (MEMs) of P with respect to T. In this paper we consider its one-pass version (Boucher et al., 2021), whose query times are dominated in our experiments by longest common extension (LCE) queries. We show how a small modification lets us avoid most of these queries and thus significantly speeds up MONI in practice while only slightly increasing its size.
- Categories:
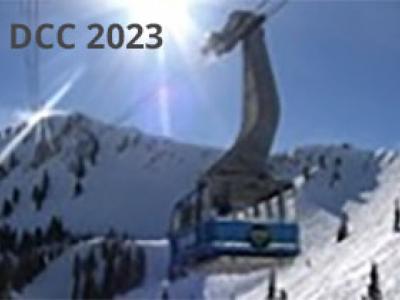
- Read more about Entropy Coding Improvement for Low-complexityCompressive Auto-encoders
- Log in to post comments
dcc_poster.pdf

- Categories:
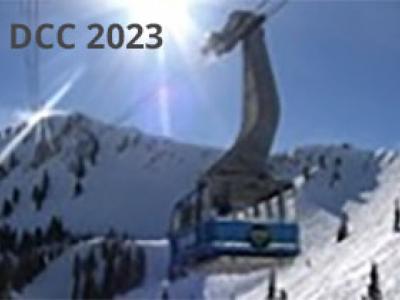
- Read more about Applicability limitations of differentiable full-reference image-quality metrics
- Log in to post comments
Subjective image-quality measurement plays a critical role in the development of image- processing applications. The purpose of a visual-quality metric is to approximate the results of subjective assessment. In this regard, more and more metrics are under development, but little research has considered their limitations. This paper addresses that deficiency: we show how image preprocessing before compression can artificially increase the quality scores provided by the popular metrics DISTS, LPIPS, HaarPSI, and VIF as well as how these scores are inconsistent with subjective-quality scores.
DCC_pptx.pptx

- Categories: