Documents
Poster
FUNCTIONAL CONNECTIVITY STATES OF THE BRAIN USING RESTRICTED BOLTZMANN MACHINES
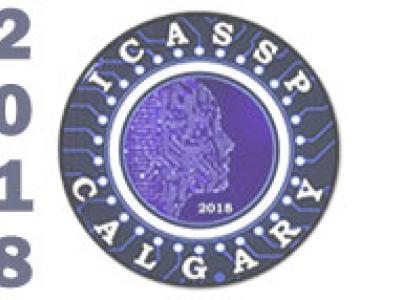
- Citation Author(s):
- Submitted by:
- Zeynep Kahraman
- Last updated:
- 14 April 2018 - 7:20am
- Document Type:
- Poster
- Document Year:
- 2018
- Event:
- Presenters:
- Zeynep Kahraman
- Paper Code:
- BISP-P3.6
- Categories:
- Log in to post comments
Recent work on resting-state functional magnetic resonance imaging (rs-fMRI) suggests that functional connectivity (FC) is dynamic. A variety of machine learning and signal processing tools have been applied to the study of dynamic functional connectivity networks (dFCNs) of the brain, by identifying a small number of network states that describe the dynamics of connectivity during rest. Recently, deep learning (DL) methods have been applied to neuroimaging data for learning generative models. In this paper, we employ the restricted Boltzmann machine (RBM), to learn FC states from resting-state dFCNs. Unlike previous applications of DL to neuroimaging data that focus on feature extraction based on the voxel level activation data, the current work employs RBM to learn connectivity patterns, where the input to RBMs are a collection of windowed covariances across time and subjects. The extracted FC states are evaluated based on their occurrence rate as well as modularity.