Documents
Poster
GaitMM: Multi-Granularity Motion Sequence Learning for Gait Recognition
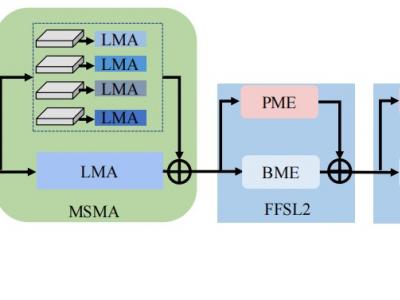
- DOI:
- 10.60864/na9h-cc80
- Citation Author(s):
- Submitted by:
- Lei Wang
- Last updated:
- 17 November 2023 - 12:05pm
- Document Type:
- Poster
- Document Year:
- 2023
- Event:
- Presenters:
- Lei Wang, Bo Liu, Bincheng Wang, Fuqiang Yu
- Paper Code:
- MP1.PB.4
- Categories:
- Log in to post comments
Gait recognition aims to identify individual-specific walking patterns by observing the different periodic movements of each body part. However, most existing methods treat each part equally and fail to account for the data redundancy caused by the different step frequencies and sampling rates of gait sequences. In this study, we propose a multi-granularity motion representation network (GaitMM) for gait sequence learning. In GaitMM, we design a combined full-body and fine-grained sequence learning module (FFSL) to explore part-independent spatio-temporal representations. Moreover, we utilize a frame-wise compression strategy, referred to as multi-scale motion aggregation (MSMA), to capture discriminative information in the gait sequence. Experiments on two public datasets, CASIA-B and OUMVLP, show that our approach reaches state-of-the-art performances.