Documents
Poster
GENEFORMER: LEARNED GENE COMPRESSION USING TRANSFORMER-BASED CONTEXT MODELING
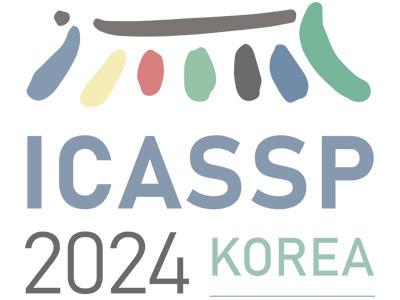
- DOI:
- 10.60864/0phz-5a35
- Citation Author(s):
- Submitted by:
- Yan Wang
- Last updated:
- 6 June 2024 - 10:55am
- Document Type:
- Poster
- Event:
- Presenters:
- Yan Wang
- Paper Code:
- MMSP-P1.10
- Categories:
- Log in to post comments
The development of gene sequencing technology sparks an explosive growth of gene data. Thus, the storage of gene data has become an important issue. Recently, researchers begin to investigate deep learning-based gene data compression, which outperforms general traditional methods. In this paper, we propose a transformer-based gene compression method named GeneFormer. Specifically, we first introduce a modified transformer encoder with latent array to eliminate the dependency of the nucleotide sequence. Then, we design a multi-level-grouping method to accelerate and improve the compression process. Experimental results on real-world datasets show that our method achieves significantly better compression ratio compared with state-of-the-art method, and the decoding speed is significantly faster than all existing learning-based gene compression methods.