Documents
Poster
Generalized Nested Latent Variable Models for Lossy Coding applied to Wind Turbine Scenarios
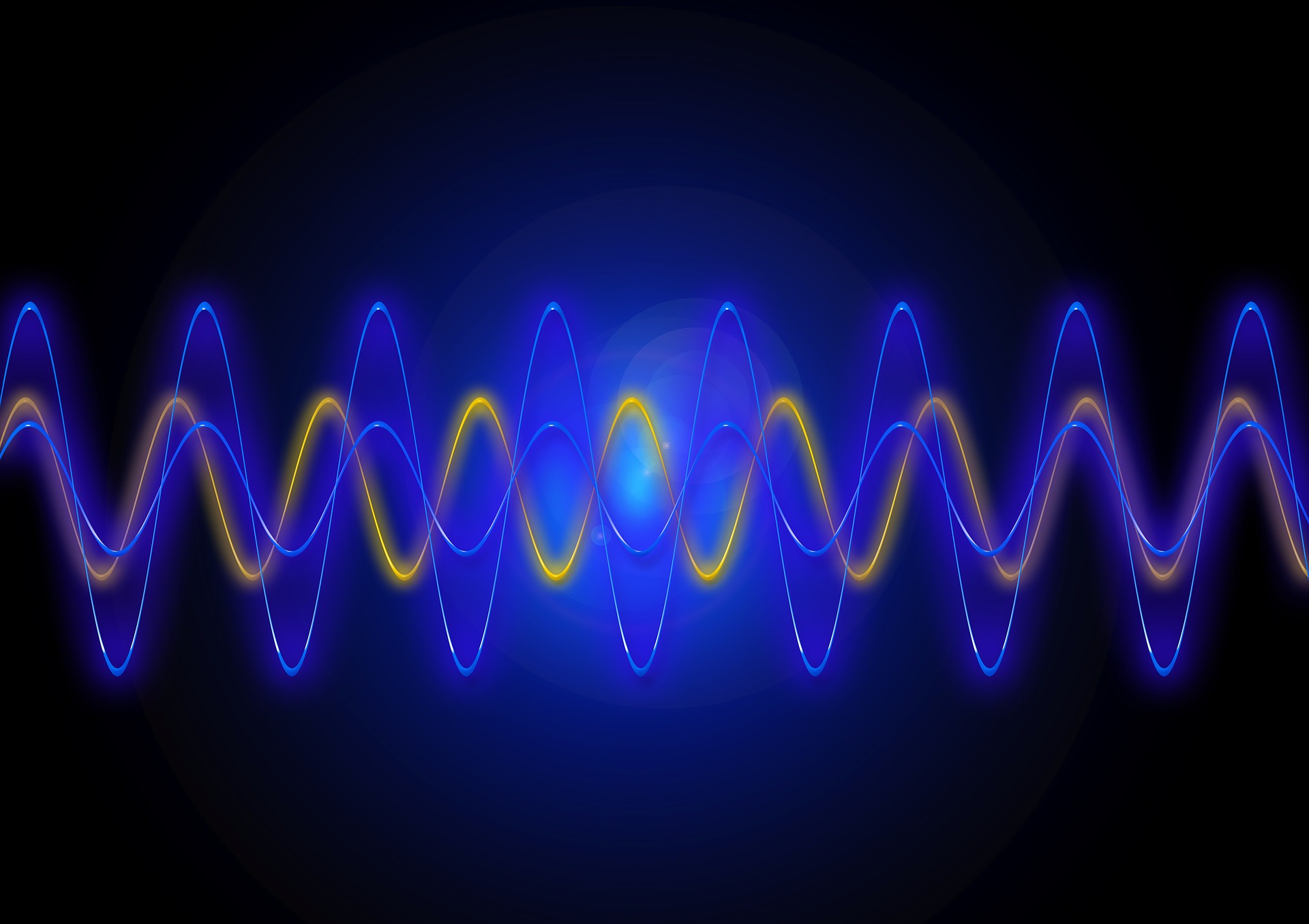
- DOI:
- 10.60864/f4rj-d061
- Citation Author(s):
- Submitted by:
- Raul Perez-Gonzalo
- Last updated:
- 27 November 2024 - 8:33am
- Document Type:
- Poster
- Categories:
- Log in to post comments
Rate-distortion optimization through neural networks has accomplished competitive results in compression efficiency and image quality. This learning-based approach seeks to minimize the compromise between compression rate and reconstructed image quality by automatically extracting and retaining crucial information, while discarding less critical details. A successful technique consists in introducing a deep hyperprior that operates within a 2-level nested latent variable model, enhancing compression by capturing complex data dependencies. This paper extends this concept by designing a generalized L-level nested generative model with a Markov chain structure. We demonstrate as L increases that a trainable prior is detrimental and explore a common dimensionality along the distinct latent variables to boost compression performance. As this structured framework can represent autoregressive coders, we outperform the hyperprior model and achieve state-of-the-art performance while reducing substantially the computational cost. Our experimental evaluation is performed on wind turbine scenarios to study its application on visual inspections.