Documents
Poster
Generative adversarial network including referring image segmentation for text-guided image manipulation
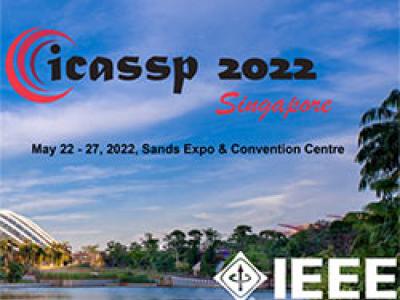
- Citation Author(s):
- Submitted by:
- Yuto Watanabe
- Last updated:
- 8 May 2022 - 4:18am
- Document Type:
- Poster
- Document Year:
- 2022
- Event:
- Presenters:
- Yuto Watanabe
- Paper Code:
- MMSP-8.1
- Categories:
- Log in to post comments
This paper proposes a novel generative adversarial network to improve the performance of image manipulation using natural language descriptions that contain desired attributes. Text-guided image manipulation aims to semantically manipulate an image aligned with the text description while preserving text-irrelevant regions. To achieve this, we newly introduce referring image segmentation into the generative adversarial network for image manipulation. The referring image segmentation aims to generate a segmentation mask that extracts the text-relevant region. By utilizing the feature map of the segmentation mask in the network, the proposed method explicitly distinguishes the text-relevant and irrelevant regions and has the following two contributions. First, our model can pay attention only to the text-relevant region and manipulate the region aligned with the text description. Second, our model can achieve an appropriate balance between the generation of accurate attributes in the text-relevant region and the reconstruction in the text-irrelevant regions. Experimental results show that the proposed method can significantly improve the performance of image manipulation.