Documents
Presentation Slides
Gradient Image Super-Resolution for Low-Resolution Image Recognition
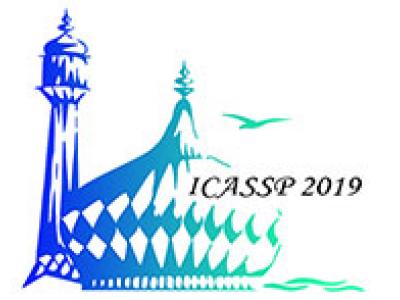
- Citation Author(s):
- Submitted by:
- Dewan fahim Noor
- Last updated:
- 10 May 2019 - 3:40pm
- Document Type:
- Presentation Slides
- Document Year:
- 2019
- Event:
- Presenters:
- Zhu Li
- Paper Code:
- 3661
- Categories:
- Log in to post comments
In visual object recognition problems essential to surveillance and navigation problems in a variety of military and civilian use cases,low-resolution and low-quality images present great challenges to this problem. Recent advancements in deep learning based methods like EDSR/VDSR have boosted pixel domain image super-resolution(SR) performances significantly in terms of signal to noise ratio(SNR)/mean square error(MSE) metrics of the super-resolved image. How-ever, these pixel domain signal quality metrics may not directly cor-relate to the machine vision tasks like key points detection and object recognition. In this work, we develop a machine vision tasks-friendly super-resolution technique which enhances the gradient images and associated features from the low-resolution images that benefit the high level machine vision tasks. Here, a residual learning deep neural network based gradient image super-resolution solution is developed with scale space adaptive network depth, and simulation results demonstrate the performance gains in both gradient image quality as well as key points repeatability