Documents
Presentation Slides
GRAPH BASED NON-UNIFORM SAMPLING AND RECONSTRUCTION OF DEPTH MAPS
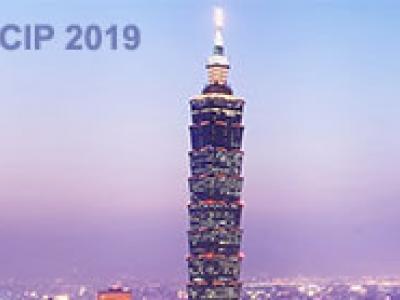
- Citation Author(s):
- Submitted by:
- Wenqiang Xu
- Last updated:
- 20 September 2019 - 3:02am
- Document Type:
- Presentation Slides
- Document Year:
- 2019
- Event:
- Presenters:
- Jingyu Yang
- Paper Code:
- 3797
- Categories:
- Log in to post comments
High-quality depth sensing is highly demanded in intelligent computer vision, 3DTV, and many other related fields. However, prevalent time-of-fly (ToF) depth sensors are of low resolution as the number of pixel-level demodulators is limited. Moreover, the rectangular sampling does not consider the signal characteristics of depth maps. Being a departure of previous resolution enhancement on rectangular sampling, this paper investigates the non-uniform sampling of depth maps, and the high-resolution depth reconstruction from limited non-uniformly distributed samples. The proposed depth sampling and reconstruction schemes are developed based on graph signal processing. We first propose a graph-based non-uniform sampling (GNS) scheme, where depth signals are sampled based on the response of a high-pass graph filter, which results in denser sampling around discontinuities such as edges and contours than in smooth regions. We then propose a graph-based depth reconstruction (GDR) framework,where a graph Laplacian regularizer is designed to fully exploit structural correlation between the depth and photometric images. To solve the reconstruction problem, we derive an efficient algorithm based on the alternating direction method of multipliers (ADMM). Experimental results show that the GNS-GDR non-uniform sampling and reconstruction method achieves high-quality depth sensing, outperforming several state-of-the-art schemes.
ICIP.ppt
