Documents
Presentation Slides
Graph Convolutional Networks with Autoencoder-Based Compression and Multi-Layer Graph Learning
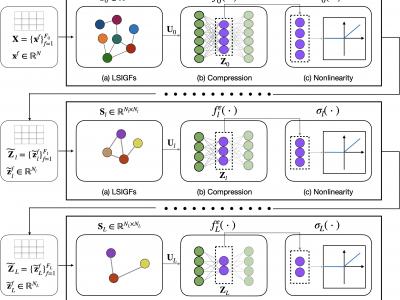
- Citation Author(s):
- Submitted by:
- Lorenzo Giusti
- Last updated:
- 8 May 2022 - 8:46am
- Document Type:
- Presentation Slides
- Event:
- Presenters:
- Lorenzo Giusti
- Categories:
- Log in to post comments
The aim of this work is to propose a novel architecture and training strategy for graph convolutional networks (GCN). The proposed architecture, named as Autoencoder-Aided GCN (AA-GCN), compresses the convolutional features in an information-rich embedding at multiple hidden layers, exploiting the presence of autoencoders before the point-wise non-linearities. Then, we propose a novel end-to-end training procedure that learns different graph representations per each layer, jointly with the GCN weights and auto-encoder parameters. As a result, the proposed strategy improves the computational scalability of the GCN, learning the best graph representations at each layer in a totally data-driven fashion. Several numerical results on synthetic and real data illustrate how our architecture and training procedure compare favorably with other state of the art solutions, both in terms of robustness and learning performance.