Documents
Presentation Slides
Graph Dictionary Learning for 3-D Point Cloud Compression
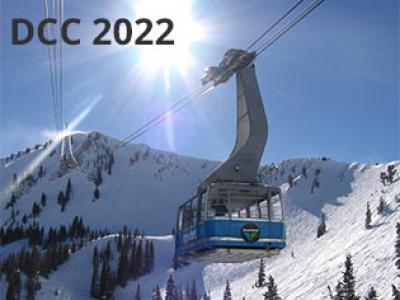
- Citation Author(s):
- Submitted by:
- Shaohui Li
- Last updated:
- 5 March 2022 - 10:21am
- Document Type:
- Presentation Slides
- Document Year:
- 2022
- Event:
- Presenters:
- Xin Li
- Paper Code:
- 216
- Categories:
- Keywords:
- Log in to post comments
3-D point clouds rendering solid representations of scenes or objects often carry a tremendous amount of points, compulsorily requesting high-efficiency compression for storage and transmission. In this paper, we propose a novel p-Laplacian embedding graph dictionary learning algorithm for 3-D point cloud attribute compression. The proposed method integrates the underlying graph topology to the learned graph dictionary capitalizing on p-Laplacian eigenfunctions and leads to parsimonious representations of 3-D point clouds. We further devise alternating optimization with the help of ADMM to efficiently solve the resulting non-convex minimization problem. Experimental results demonstrate that the proposed method outperforms state-of-the-art and recent transform-based methods in 3-D point cloud compression.