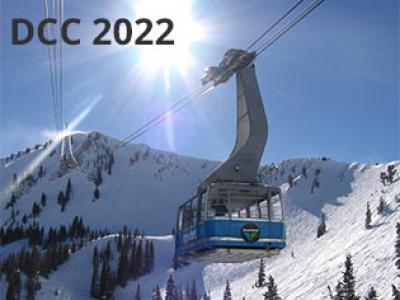
3-D point clouds rendering solid representations of scenes or objects often carry a tremendous amount of points, compulsorily requesting high-efficiency compression for storage and transmission. In this paper, we propose a novel p-Laplacian embedding graph dictionary learning algorithm for 3-D point cloud attribute compression. The proposed method integrates the underlying graph topology to the learned graph dictionary capitalizing on p-Laplacian eigenfunctions and leads to parsimonious representations of 3-D point clouds.
- Categories: