Documents
Presentation Slides
GRAPH METRIC LEARNING VIA GERSHGORIN DISC ALIGNMENT
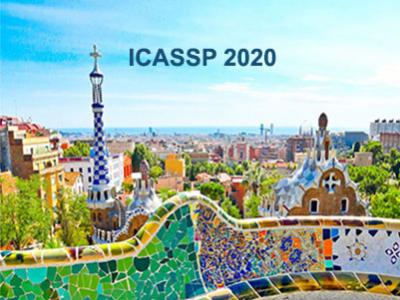
- Citation Author(s):
- Submitted by:
- CHENG YANG
- Last updated:
- 15 May 2020 - 4:01pm
- Document Type:
- Presentation Slides
- Document Year:
- 2020
- Event:
- Presenters:
- Gene Cheung
- Paper Code:
- ICASSP 2020 Paper #2062 Paper ID: TU2.PG.2
- Categories:
- Log in to post comments
We propose a general projection-free metric learning framework, where the minimization objective $\min_{\M \in \cS} Q(\M)$ is a convex differentiable function of the metric matrix $\M$, and $\M$ resides in the set $\cS$ of generalized graph Laplacian matrices for connected graphs with positive edge weights and node degrees.
Unlike low-rank metric matrices common in the literature, $\cS$ includes the important positive-diagonal-only matrices as a special case in the limit.
The key idea for fast optimization is to rewrite the positive definite cone constraint in $\cS$ as signal-adaptive linear constraints via Gershgorin disc alignment, so that the alternating optimization of the diagonal and off-diagonal terms in $\M$ can be solved efficiently as linear programs via Frank-Wolfe iterations.
We prove that left-ends of the Gershgorin discs can be aligned perfectly using the first eigenvector $\v$ of $\M$, which we update iteratively using Locally Optimal Block Preconditioned Conjugate Gradient (LOBPCG) with warm start as diagonal / off-diagonal terms are optimized.
Experiments show that our efficiently computed graph metric matrices outperform metrics learned using competing methods in terms of classification tasks.