Documents
Presentation Slides
HACD: Hierarchical Agglomerative Community Detection in Social Networks
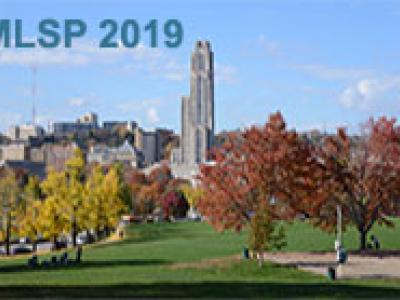
- Citation Author(s):
- Submitted by:
- Ekta Gujral
- Last updated:
- 12 October 2019 - 5:58pm
- Document Type:
- Presentation Slides
- Document Year:
- 2019
- Event:
- Presenters:
- Evangelos E. Papalexakis
- Categories:
- Log in to post comments
Communities (also referred to as clusters) are essential building blocks of all networks. Hierarchical clustering methods are common graph-based approaches for graph clustering. Traditional hierarchical clustering algorithms proceed in a bottom-up or top-down fashion to encode global information in the graph and cluster according to the global modularity of the graph. In this paper, we propose an efficient Hierarchical Agglomerative Community Detection (HACD) algorithm, that combines the local information in a graph with membership propagation to solve the problem, achieving 10-25% quality improvement overall baselines. The first contribution of this paper is to present the fundamental limitations of the general modularity optimization-based approach. We show that based only on modularity information, the method does not provide high-quality clusters. Furthermore, even with modularity optimization, we experimentally show that the final level partitioning of such methods cannot successfully cluster data that contain highly mixed structures at different levels and densities. Based on these findings, the second contribution of this paper is a novel method to propagate knowledge throughout the graph, to split or merge the communities in order to evaluate the consistency of individual clusters. Our approach is bottom-up graph-based clustering, is scale-free, and can determine clusters at all scales. We extensively evaluate HACD’s performance in comparison to state-of-the-art approaches across six real-world and seven synthetic benchmark datasets and demonstrate that HACD, through combining graph’s local information and membership propagation, outperforms the baselines in terms of finding well-integrated communities.