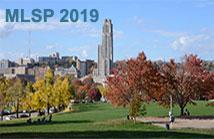
The 29th MLSP workshop in the series of workshops organized by the IEEE Signal Processing Society MLSP Technical Committee will take place at the University of Pittsburgh Campus, Pittsburgh, PA, USA and present the most recent and exciting advances in machine learning for signal processing through keynote talks, tutorials, special and regular single-track sessions as well as matchmaking events.
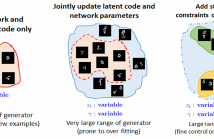
- Read more about GENERATIVE MODELS FOR LOW-RANK VIDEO REPRESENTATION AND RECONSTRUCTION FROM COMPRESSIVE MEASUREMENTS
- Log in to post comments
Generative models have recently received considerable attention in the field of compressive sensing. If an image belongs to the range of a pretrained generative network, we can recover it from its compressive measurements by estimating the underlying compact latent code. In practice, all the pretrained generators have certain range beyond which they fail to generate reliably. Recent researches show that convolutional generative structures are biased to generate natural images.
- Categories:
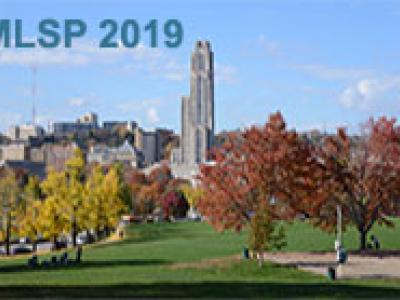
- Read more about A Multimodal Dense U-Net for Accelerating Multiple Sclerosis MRI
- Log in to post comments
The clinical analysis of magnetic resonance (MR) can be accelerated through the undersampling in the k-space (Fourier domain). Deep learning techniques have been recently received considerable interest for accelerating MR imaging (MRI). In this paper, a deep learning method for accelerating MRI is presented, which is able to reconstruct undersampled MR images obtained by reducing the k-space data in the direction of the phase encoding.
MLSP2019_poster_v4.pdf

- Categories:
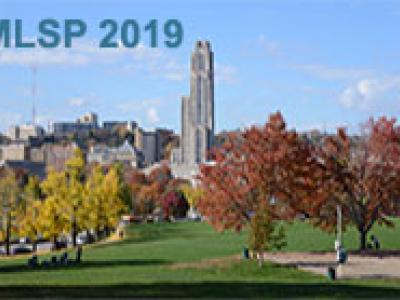
- Read more about End-to-end Detection of Attacks to Automatic Speaker Recognizers with Time-attentive Light Convolutional Neural Networks
- Log in to post comments
In this contribution, we introduce convolutional neural network architectures aiming at performing end-to-end detection of attacks to voice biometrics systems, i.e. the model provides scores corresponding to the likelihood of attack given general purpose time-frequency features obtained from speech. Microphone level attackers based on speech synthesis and voice conversion techniques are considered, along with presentation replay attacks.
- Categories:
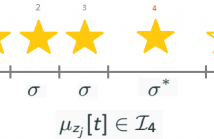
- Read more about A WEIGHTED ORDERED PROBIT COLLABORATIVE KALMAN FILTER FOR HOTEL RATING PREDICTION
- Log in to post comments
A successful recommender system interacts with users and learns their preferences. This is crucial in order to provide accurate recommendations. In this paper, a Weighted Ordered Probit Collaborative Kalman filter is proposed for hotel rating prediction. Since potential changes may occur in hotel services or accommodation conditions, a hotel popularity may be volatile through time. A weighted ordered probit model is introduced to capture this latent trend about each hotel popularity through time.
- Categories:
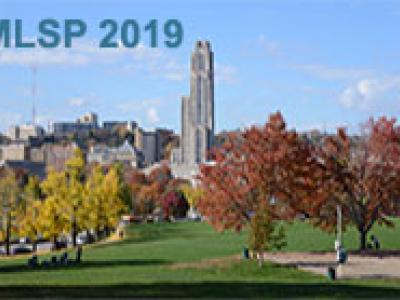
- Read more about Efficient Capon-based approach exploiting temporal windowing for electric network frequency estimation
- Log in to post comments
Electric Network Frequency (ENF) fluctuations constitute a powerful tool in multimedia forensics. An efficient approach for ENF estimation is introduced with temporal windowing based on the filter-bank Capon spectral estimator. A type of Gohberg-Semencul factorization of the model covariance matrix is used due to the Toeplitz structure of the covariance matrix. Moreover, this approach uses, for the first time in the field of ENF, a temporal window, not necessarily the rectangular one, at the stage preceding spectral estimation.
poster2019.pdf

- Categories:
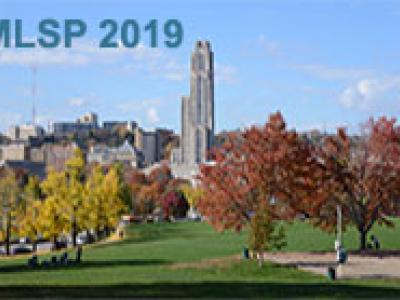
- Read more about Insights into the behaviour of multi-task deep neural networks for medical image segmentation
- Log in to post comments
Glandular morphology is used by pathologists to assess the malignancy of different adenocarcinomas. This process involves conducting gland segmentation task. The common approach in specialised domains, such as medical imaging, is to design complex architectures in a multi-task learning setup. Generally, these approaches rely on substantial postprocessing efforts. Moreover, a predominant notion is that general purpose models are not suitable for gland instance segmentation. We analyse the behaviour of two architectures: SA-FCN and Mask R-CNN.
- Categories:
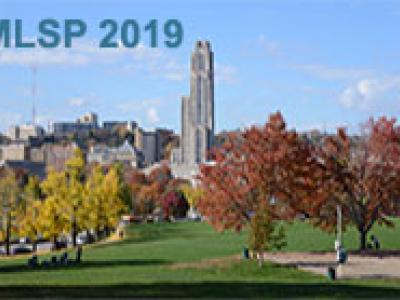
- Read more about A deep network for single-snapshot direction of arrival estimation
- Log in to post comments
This paper examines a deep feedforward network for beamforming with the single--snapshot Sample Covariance Matrix (SCM). The Conventional beamforming formulation, typically quadratic in the complex weight space, is reformulated as real and linear in the weight covariance and SCM. The reformulated SCMs are used as input to a deep feed--forward neural network (FNN) for two source localization. Simulations demonstrate the effect of source incoherence and performance in a noisy tracking example.
- Categories:
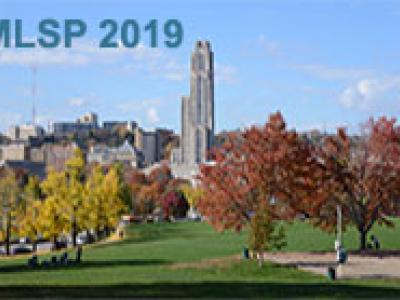
- Read more about On Convergence of Projected Gradient Descent for Minimizing a Large Scale Quadratic over the Unit Sphere
- Log in to post comments
Unit sphere-constrained quadratic optimization has been studied extensively over the past decades. While state-of-art algorithms for solving this problem often rely on relaxation or approximation techniques, there has been little research into scalable first-order methods that tackle the problem in its original form. These first-order methods are often more well-suited for the big data setting. In this paper, we provide a novel analysis of the simple projected gradient descent method for minimizing a quadratic over a sphere.
MLSP2019.pdf

- Categories:
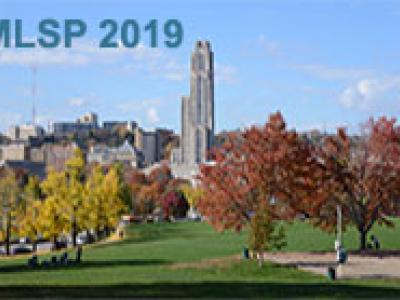
- Read more about Efficient Parameter Estimation for Semi-Continuous Data: An Application to Independent Component Analysis
- Log in to post comments
MLSP_2019.pdf

- Categories:
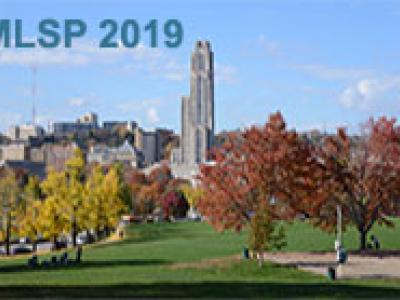