Documents
Poster
HAZY REMOTE SENSING IMAGES SEMANTIC SEGMENTATION FOR WEAKLY ANNOTATION BASED ON SALIENCY-AWARE ALIGNMENT STRATEGY
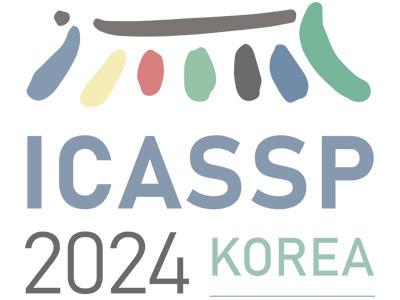
- DOI:
- 10.60864/564q-cf66
- Citation Author(s):
- Submitted by:
- Junda Xu
- Last updated:
- 5 April 2024 - 4:28am
- Document Type:
- Poster
- Event:
- Categories:
- Log in to post comments
The technique of semantic segmentation (SS) holds significant importance in the domain of remote sensing image (RSI) processing. The current research primarily encompasses two problems: 1) RSIs are easily affected by clouds and haze; 2) SS based on strong annotation requires vast human and time costs. In this paper, we propose a weakly supervised semantic segmentation (WSSS) method for hazy RSIs based on saliency-aware alignment strategy. Firstly, we design alignment network (AN) and target network (TN) with the same structure. After training the AN with clear images, we extract the class activation maps of the two networks and construct a consistency loss to train the TN with hazy images. Secondly, we design a multi-scale channel-spatial attention module in the two classification networks to solve the problems of unclear foreground-background boundary and blurred target texture in hazy images. Finally, the pseudo-labels generated by the TN are utilized to train a feedback saliency analysis network, which is subsequently employed for obtaining segmentation results during the testing phase. The experiment results demonstrate that our approach achieves superior SS performance for hazy RSIs.
Comments
1
1