Documents
Poster
A HYBRID PRIOR MODEL FOR TUNABLE DIODE LASER ABSORPTION TOMOGRAPHY
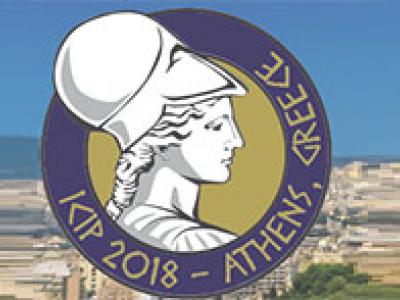
- Citation Author(s):
- Submitted by:
- Zeeshan Nadir
- Last updated:
- 6 October 2018 - 5:02pm
- Document Type:
- Poster
- Document Year:
- 2018
- Event:
- Presenters:
- Zeeshan Nadir
- Paper Code:
- MA.P4.6
- Categories:
- Keywords:
- Log in to post comments
Model based methods have gained popularity in the past few decades in reconstruction problems particularly when the measurement data is sparse. In model based inference, apart from a model for the measurements, there exists a model for the unknown signal to be reconstructed, called the prior model. Model based methods tend to do very well when the prior model is accurate and representative of real world behavior of the unknown signal. Often these priors are trained from some training data, and therefore, the accuracy of the reconstructions depends inherently on the accuracy of the training data. The reconstructions can come out to be highly biased if the training data is not representative of the actual signal. In this paper, we propose a new hybrid prior model that combines a Markov Random Field model with a Gaussian model trained from a sparse training set. We combine the models using a mixing coefficient γ ∈ [0, 1], that controls the influence of each of the models. Our main contribution is in the way we combine the two models to produce a whole continuum of prior models for different values of γ, where γ can be tuned according to how trustworthy the training set is. Reconstruction results show that our hybrid prior models produces high quality reconstructions even when the training data is not representative.