Documents
Presentation Slides
Hyperspectral remote sensing data compression with neural networks
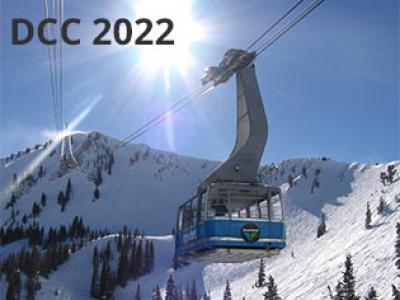
- Citation Author(s):
- Submitted by:
- Sebastia Mijare...
- Last updated:
- 3 March 2022 - 2:54pm
- Document Type:
- Presentation Slides
- Document Year:
- 2022
- Event:
- Presenters:
- Sebastia Mijares i Verdu
- Categories:
- Log in to post comments
We propose a novel approach to compress hyperspectral remote sensing images using convo- lutional neural networks, aimed at producing compression results competitive with common lossy compression standards such as JPEG 2000 and CCSDS 122.1-B-1 with a system far less complex than equivalent neural-network codecs used for natural images. Our method consists of a collection of smaller networks which compress the image band-by-band taking advantage of the very high similarity between bands on certain intervals. This approach is far less computationally complex than using a conventional neural-network codec, and we show it is effective on AVIRIS images, where we trained models that can match or surpass JPEG 2000 by around 1.5 dB at rates below 0.15 bps for uncalibrated data, and which surpass CCSDS 122.1-B-1 by up to around 5 dB across all rates.