Documents
Presentation Slides
ICASSP 2019 presentation slides
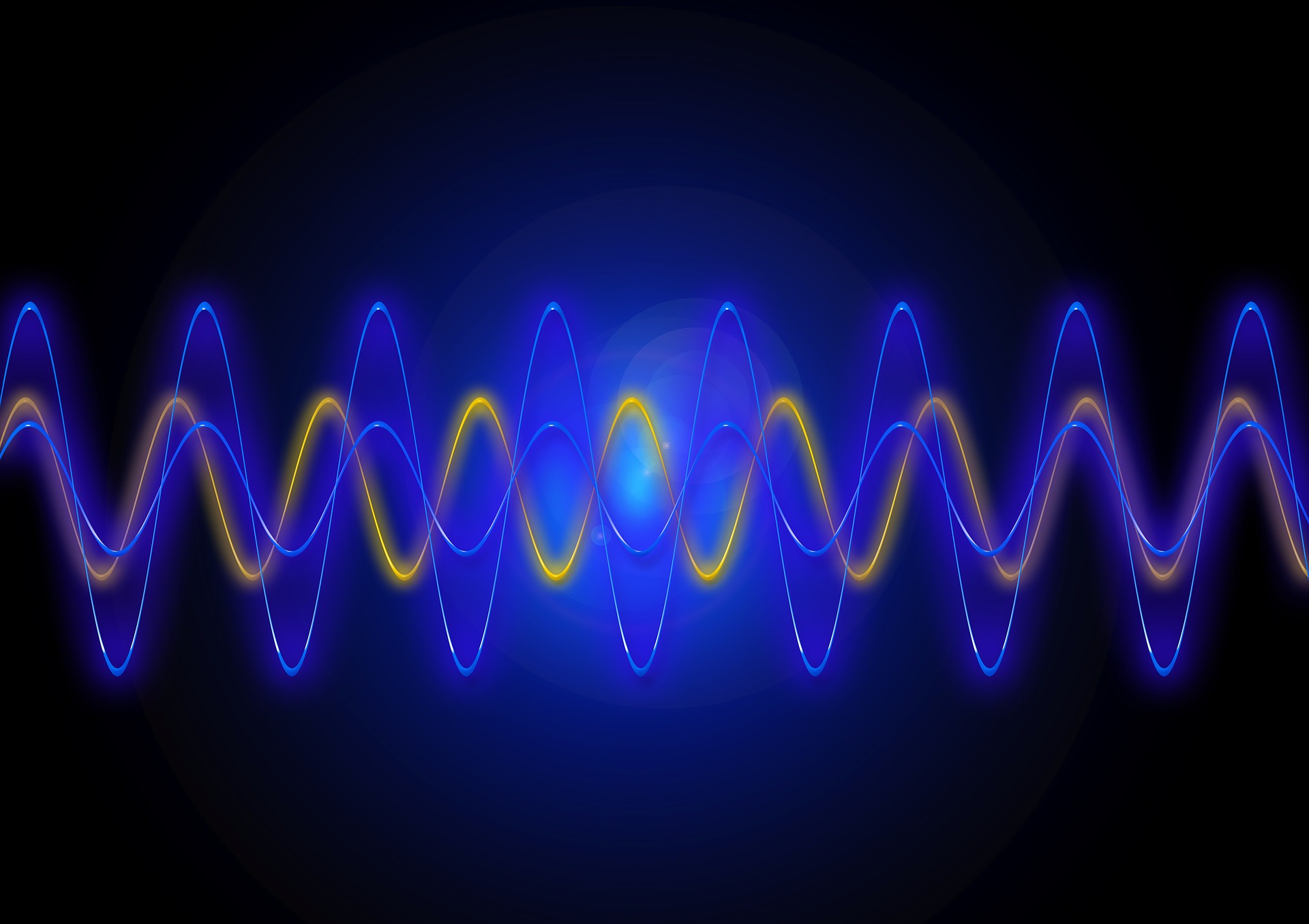
- Citation Author(s):
- Submitted by:
- Lukas Pfeifenberger
- Last updated:
- 28 April 2021 - 6:26am
- Document Type:
- Presentation Slides
- Document Year:
- 2019
- Presenters:
- Lukas Pfeifenberger
- Paper Code:
- 2996
- Categories:
- Log in to post comments
We propose a complex-valued deep neural network (cDNN) for speech enhancement and source separation. While existing end-to-end systems use complex-valued gradients to pass the training error to a real-valued DNN used for gain mask estimation, we use the full potential of complex-valued LSTMs, MLPs and activation functions to estimate complex-valued beamforming weights directly from complex-valued microphone array data. By doing so, our cDNN is able to locate and track different moving sources by exploiting the phase information in the data. In our experiments, we use a typical living room environment, mixtures of the WallStreet Journal corpus, and YouTube noise. We compare our cDNN against the BeamformIt toolkit as a baseline, and a mask-based beamformer as a state-of-the-art reference system. We observed a significant improvement in terms of PESQ, STOI and WER.