Documents
ICIP2025_Supplementary Materials
ICIP2025_Sparse_3D_Suppl
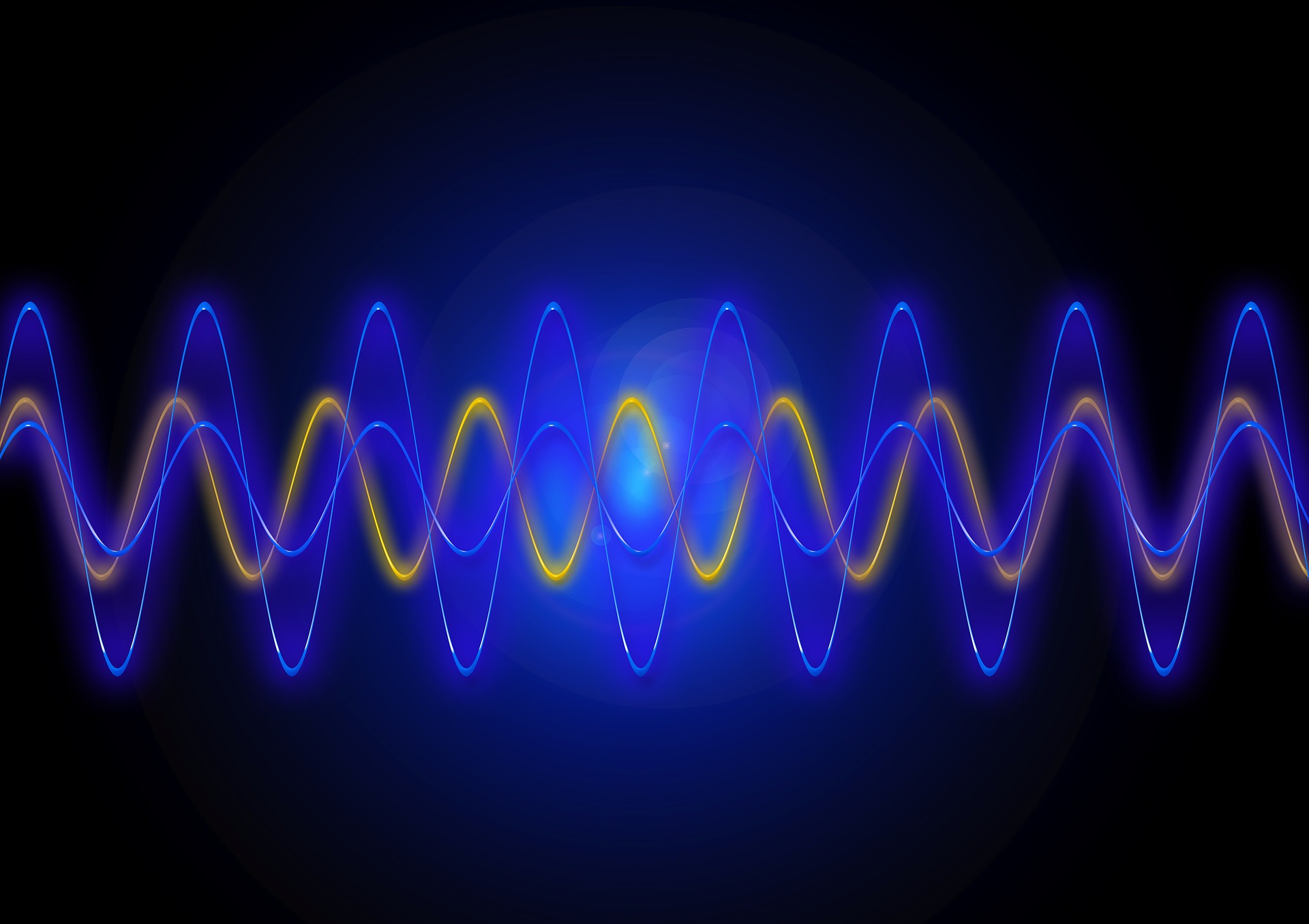
- DOI:
- 10.60864/sw3b-1w78
- Citation Author(s):
- Submitted by:
- Guangan Chen
- Last updated:
- 31 December 2024 - 3:39pm
- Document Type:
- ICIP2025_Supplementary Materials
- Categories:
- Log in to post comments
Novel-view synthesis in 360$^\circ$ scenes from an extremely sparse set of input views is essential for applications like virtual reality and augmented reality, where using fewer views reduces costs and simplifies setup.
However, sparse input views introduce significant challenges: 1) typical structure-from-motion methods often fail to estimate camera poses, 2) geometry and appearance of scenes may only be partially reconstructed, and 3) generated novel views may exhibit inconsistencies, such as distorted shapes or varying object appearances, caused by incomplete visual input.
To address these challenges, this paper presents a novel framework tailored for 3D Gaussian Splatting in extremely sparse-view cases. Specifically, we apply DUSt3R to estimate camera poses and generate a dense point cloud as input. We strategically sample additional views in the upper hemisphere space of the scenes and create supplementary training data by rendering synthetic images with the point cloud. To improve the quality of the synthetic images, we retrain a diffusion-based image enhancement model using our created dataset to remove point-cloud rendering artifacts.
We validate our framework against related benchmarks for cases with only four input views, demonstrating significant improvements in the fidelity of novel-view synthesis outputs under extremely sparse-view conditions.