Documents
Supplementary
ICIP2025_SUPPLEMENTARY_MODEL_ATTACK
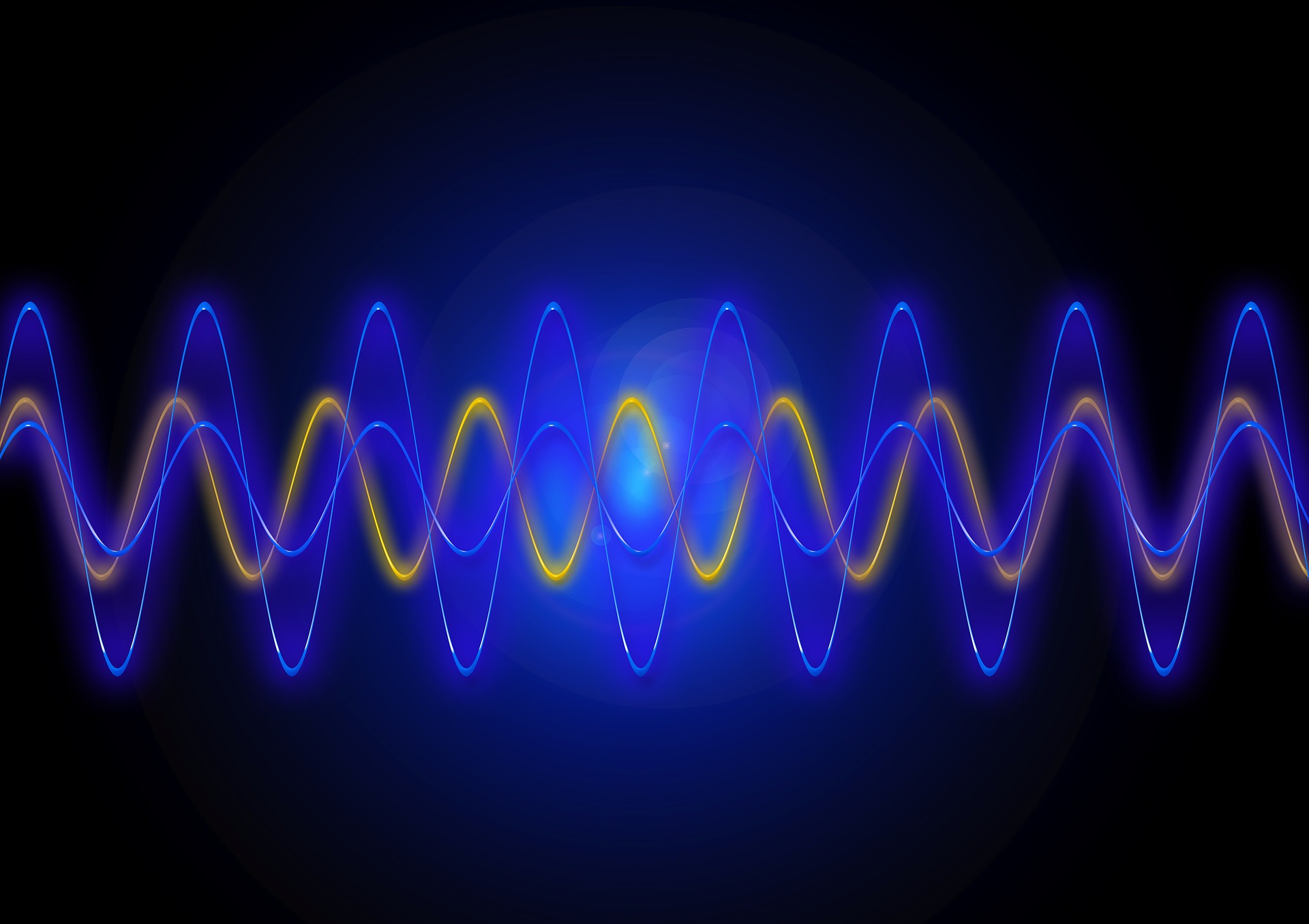
- DOI:
- 10.60864/xtc1-sw37
- Citation Author(s):
- Submitted by:
- Anonymous for s...
- Last updated:
- 5 February 2025 - 8:33pm
- Document Type:
- Supplementary
- Categories:
- Log in to post comments
Recent advancements in diffusion models have enabled high-fidelity and photorealistic image generation across diverse applications. However, these models also present security and privacy risks, including copyright violations, sensitive information leakage, and the creation of harmful or offensive content that could be exploited maliciously. In this study, we uncover a novel security threat where an attacker leverages diffusion model APIs to generate synthetic images, which are then used to train a high-performing substitute model. This enables the attacker to execute model extraction and transfer-based adversarial attacks on black-box classification models with minimal queries, without needing access to the original training data. The generated images are sufficiently high-resolution and diverse to train a substitute model whose outputs closely match those of the target model. Across the seven benchmarks, including CIFAR and ImageNet subsets, our method shows an average improvement of 27.37% over state-of-the-art methods while using just 0.01times of the query budget, achieving a 98.68% success rate in adversarial attacks on the target model.