Documents
Poster
Improved Data Selection for Domain Adaptation in ASR
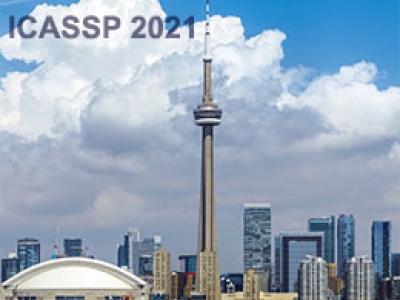
- Citation Author(s):
- Submitted by:
- Shannon Wotherspoon
- Last updated:
- 25 June 2021 - 2:45pm
- Document Type:
- Poster
- Document Year:
- 2021
- Event:
- Presenters:
- Shannon Wotherspoon
- Paper Code:
- 4438
- Categories:
- Log in to post comments
Automatic speech recognition (ASR) systems are highly sensitive to train-test domain mismatch. However, because transcription is often prohibitively expensive, it is important to be able to make use of available transcribed out-of-domain data. We address the problem of domain adaptation with semi-supervised training (SST). Contrary to work in in-domain SST, we find significant performance improvement even with just one hour of target-domain data—though, the selection of the data is critical. We show that minimum phone error rate is a good oracle measure for selection, and we approximate this measure by using the average phone confidence of an utterance. With larger domain shifts, we also find that deletions and low lexical diversity are a serious issue, which we address by incorporating phone rate into our selection metric. With our proposed selection criterion, we see up to 57% relative improvements over the out-of-domain baseline model. Furthermore, this selection method generalizes well, and matches or outperforms word-level confidence selection across six separate domain shift conditions.