Documents
Poster
Improving Content-based Audio Retrieval by Vocal Imitation Feedback
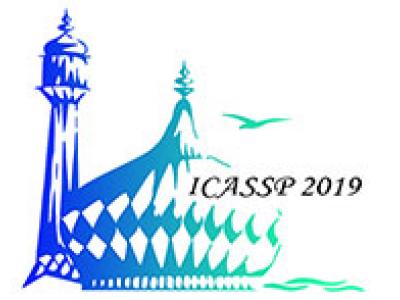
- Citation Author(s):
- Submitted by:
- Bongjun Kim
- Last updated:
- 11 May 2019 - 1:06am
- Document Type:
- Poster
- Document Year:
- 2019
- Event:
- Presenters:
- Bongjun Kim
- Paper Code:
- 2611
- Categories:
- Log in to post comments
Content-based audio retrieval including query-by-example (QBE) and query-by-vocal imitation (QBV) is useful when search-relevant text labels for the audio are unavailable, or text labels do not sufficiently narrow the search. However, a single query example may not provide sufficient information to ensure the target sound(s) in the database are the most highly ranked. In this paper, we adapt an existing model for generating audio embeddings to create a state-of-the-art similarity measure for audio QBE and QBV. We then propose a new method to update search results when top-ranked items are not relevant: The user provides an additional vocal imitation to illustrate what they do or do not want in the search results. This imitation may either be of some portion of the initial query example, or of a top-ranked (but incorrect) search result. Results show that adding vocal imitation feedback improves initial retrieval results by a statistically significant amount.