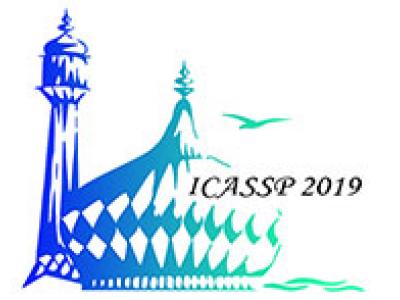
- Read more about Improving Content-based Audio Retrieval by Vocal Imitation Feedback
- Log in to post comments
Content-based audio retrieval including query-by-example (QBE) and query-by-vocal imitation (QBV) is useful when search-relevant text labels for the audio are unavailable, or text labels do not sufficiently narrow the search. However, a single query example may not provide sufficient information to ensure the target sound(s) in the database are the most highly ranked. In this paper, we adapt an existing model for generating audio embeddings to create a state-of-the-art similarity measure for audio QBE and QBV.
- Categories:
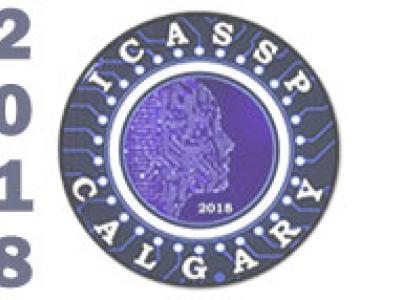
- Read more about Framework For Evaluation Of Sound Event Detection In Web Videos
- Log in to post comments
The largest source of sound events is web videos. Most videos lack sound event labels at segment level, however, a significant number of them do respond to text queries, from a match found using metadata by search engines. In this paper we explore the extent to which a search query can be used as the true label for detection of sound events in videos. We present a framework for large-scale sound event recognition on web videos. The framework crawls videos using search queries corresponding to 78 sound event labels drawn from three datasets.
- Categories:
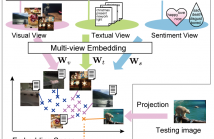
- Read more about IMAGE SENTIMENT ANALYSIS USING LATENT CORRELATIONS AMONG VISUAL, TEXTUAL, AND SENTIMENT VIEWS
- Log in to post comments
As Internet users increasingly post images to express their daily sentiment and emotions, the analysis of sentiments in user-generated images is of increasing importance for developing several applications. Most conventional methods of image sentiment analysis focus on the design of visual features, and the use of text associated to the images has not been sufficiently investigated. This paper proposes a novel approach that exploits latent correlations among multiple views: visual and textual views, and a sentiment view constructed using SentiWordNet.
- Categories: