Documents
Presentation Slides
Improving Visual Quality and Transferability of Adversarial Attacks on Face Recognition Simultaneously with Adversarial Restoration
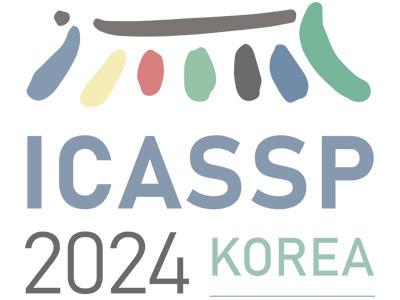
- Citation Author(s):
- Submitted by:
- Fengfan Zhou
- Last updated:
- 8 April 2024 - 6:38am
- Document Type:
- Presentation Slides
- Event:
- Categories:
- Log in to post comments
Adversarial face examples possess two critical properties: Visual Quality and Transferability. However, existing approaches rarely address these properties simultaneously, leading to subpar results. To address this issue, we propose a novel adversarial attack technique known as Adversarial Restoration (AdvRestore), which enhances both visual quality and transferability of adversarial face examples by leveraging a face restoration prior. In our approach, we initially train a Restoration Latent Diffusion Model (RLDM) designed for face restoration. Subsequently, we employ the inference process of RLDM to generate adversarial face examples. The adversarial perturbations are applied to the intermediate features of RLDM. Additionally, by treating RLDM face restoration as a sibling task, the transferability of the generated adversarial face examples is further improved. Our experimental results validate the effectiveness of the proposed attack method.
slide.pptx
